论文地址:Language-Agnostic Representation Learning of Source Code from Structure and Context
CodeTransformer:只使用AST语言无关特征做多语言训练
Abstract
原代码(context)和语法结构树(AST)是程序的两种互补表示,本文模型只使用了语言无关的特征,即原代码和特征可以直接从AST中计算得到。除了在五种编程语言单语言代码摘要SOTA外,也是第一个提出了多语言代码摘要模型,对来自多种编程语言的非并行数据进行联合训练可以提高所有单个语言的结果,特别是在低资源的语言上。只在context多语言训练不能得到同样的提升,所以结果肯定是结构和上下文组合得到的
Introduction
研究人员和从业者在机器学习模型中主要利用结构或上下文,作者证明了上下文和结构的联合学习改进了源代码的表示学习
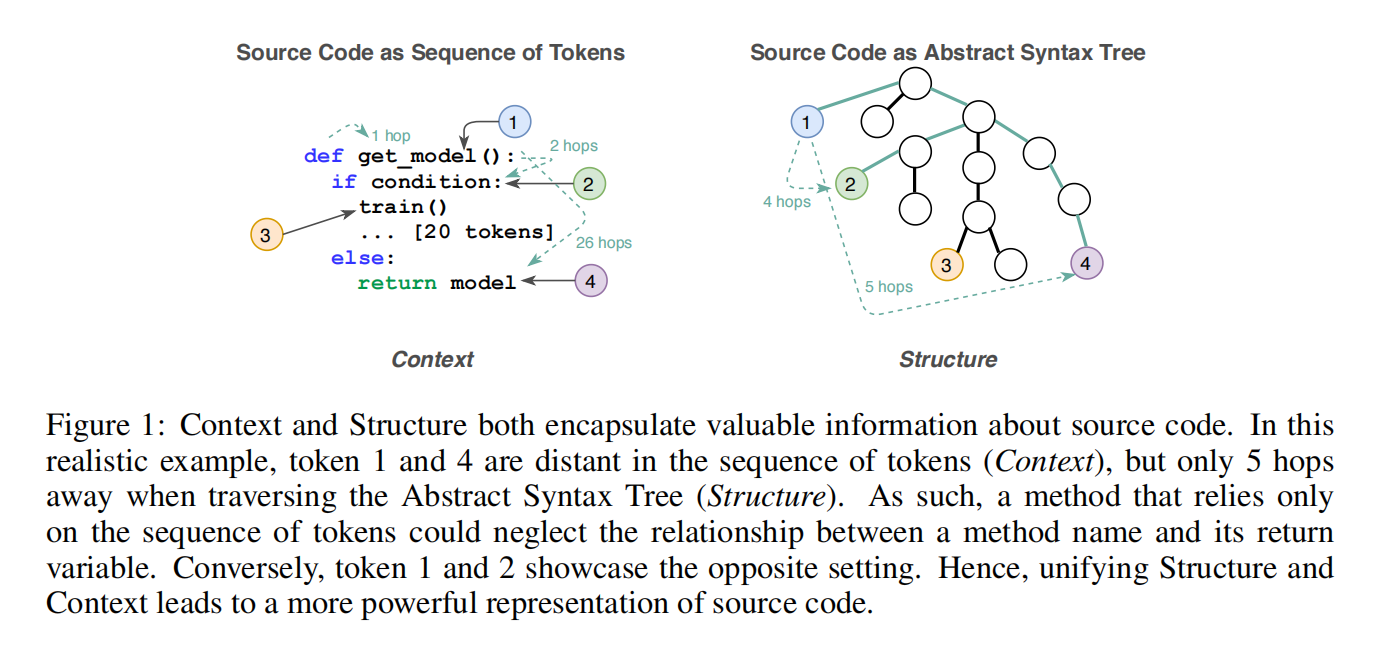
作者提出了CodeTransformer结合了在自注意操作在结构和上下文上计算的距离,与通过边进行的局部处理相比,计算AST上的成对距离,如最短路径长度,使每一层的模型都可以访问完整的结构。此外在注意力计算时使用了相对距离替代绝对位置,这些都是语言无关的特征可以直接使用
Integrating Structure and Context in the Code Transformer
Integrating Source Code and AST Representations of Programs
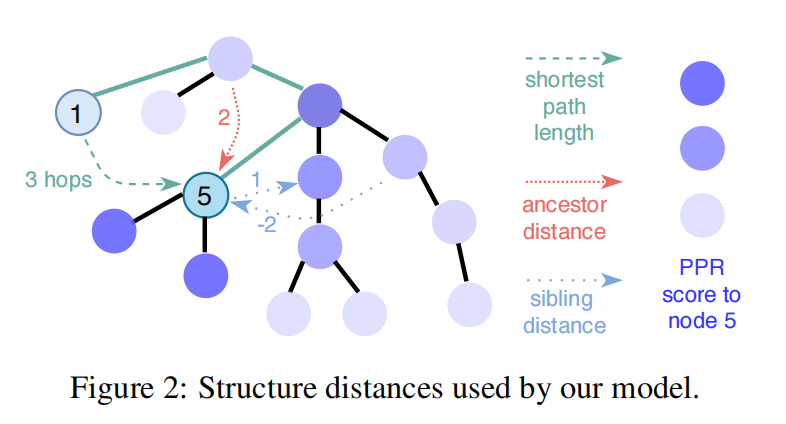
PPR是Personalized PageRank
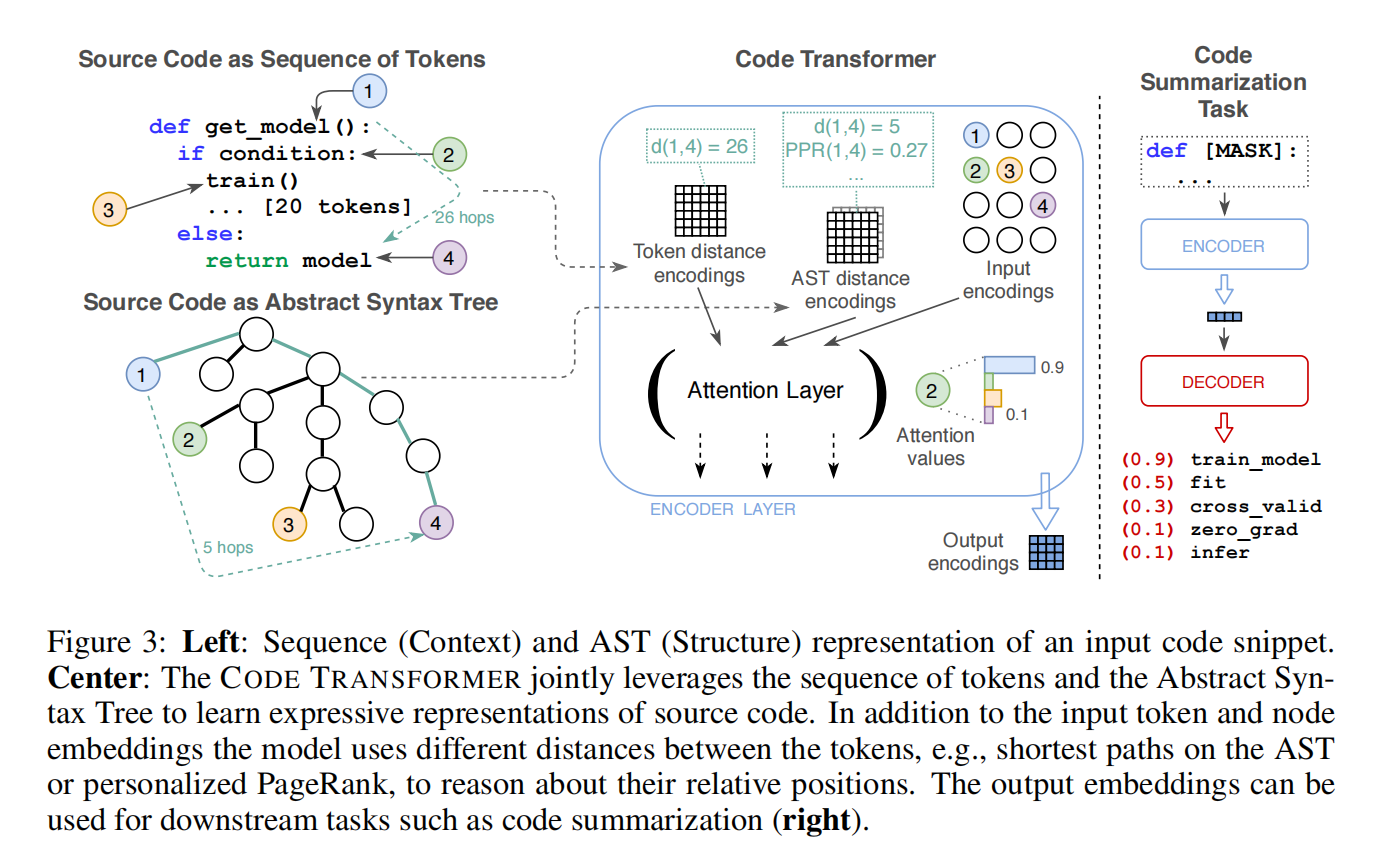
Efficient Relative Attention Computation

这里用的解耦注意力和相对位置距离
Experiment
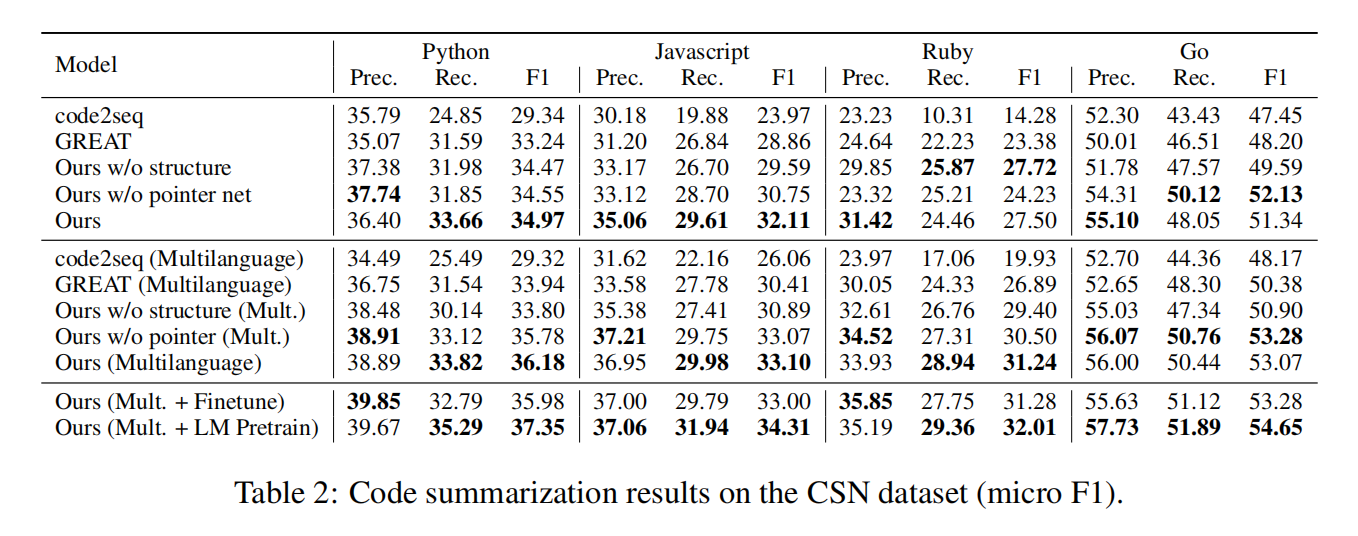
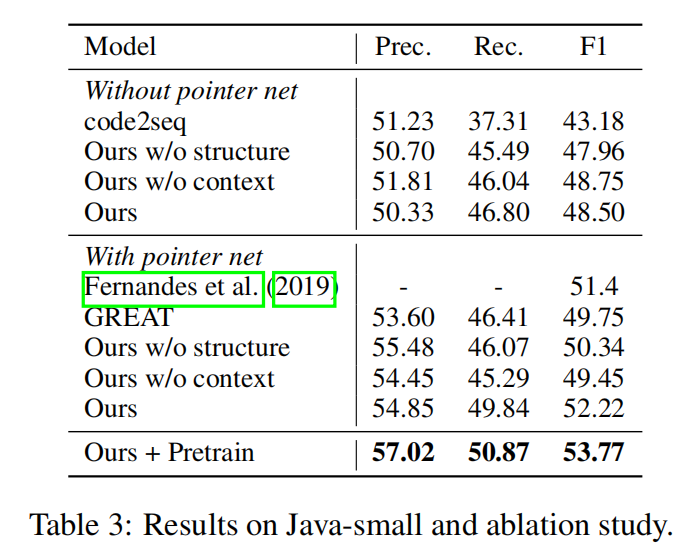
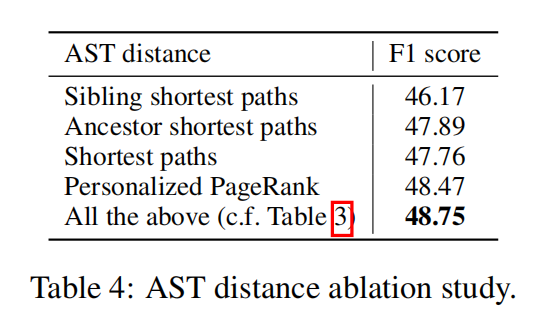