论文地址:Automatic source code summarization with graph attention networks
GSCS:语义和结构两个encoder融合
Abstract
本文提出了GSCS利用了代码块的语义和结构信息,GAT处理AST,使用了多头注意力;还使用了RNN模型来获取语义特征
Introduction
源代码有复杂的嵌套结构,不能视作朴素文本;现有模型也不能很好的处理结构信息,比如SBT引入了大量的括号造成不必要的冗余,Tree-LSTM和ConvGNN没有考虑到AST节点的上下文,换句话说每个节点在AST里面都是有局部属性的,但上述两种方法是不考虑的(a+b和b+a是同等对待)
作者认为使用GAT能自动学习并分配权重给邻居,是比Tree-LSTM和ConvGNN更好的选择
Approach
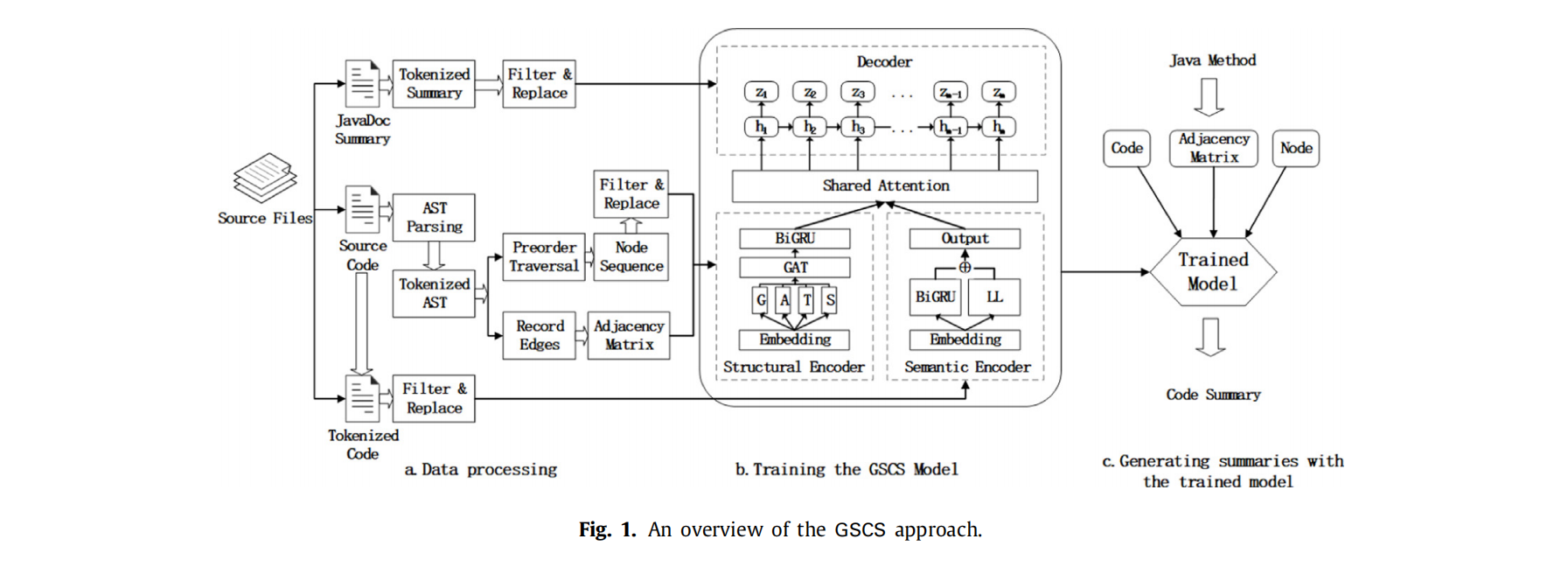
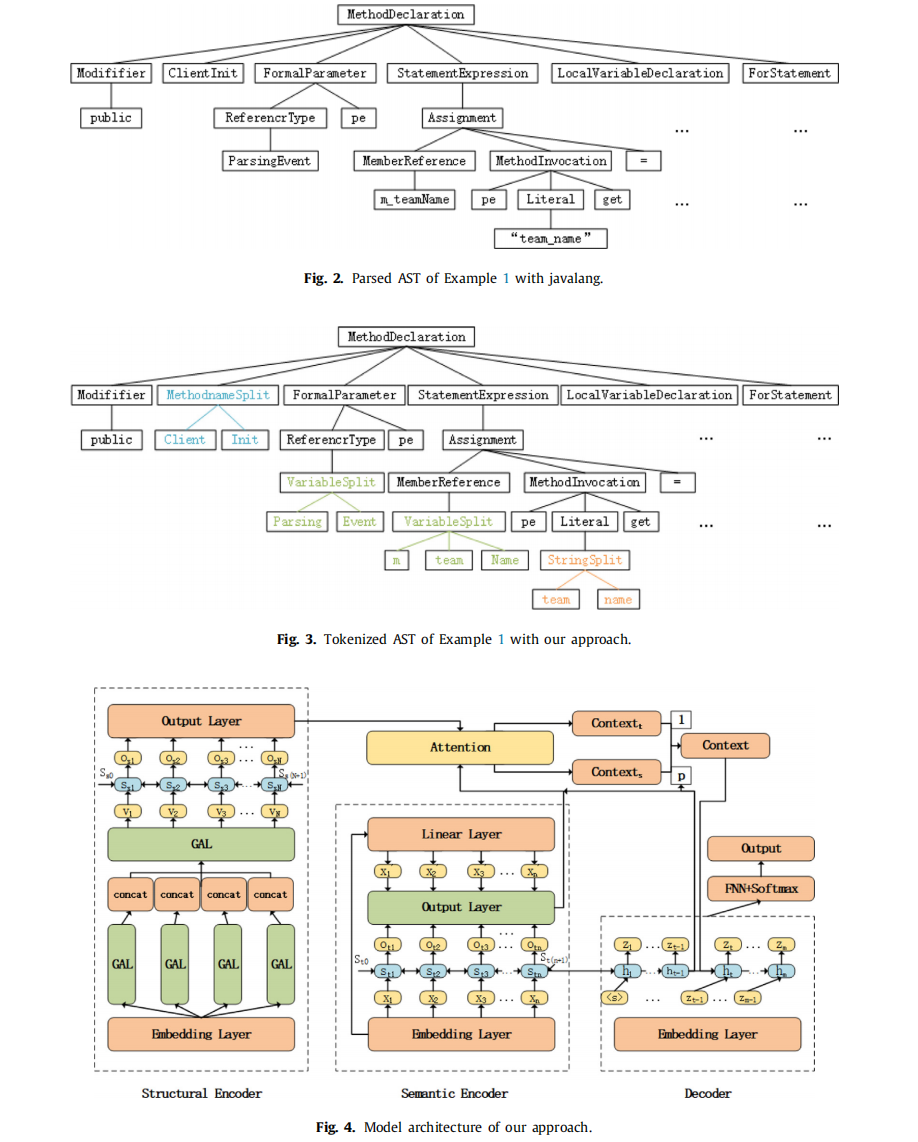
Evaluation
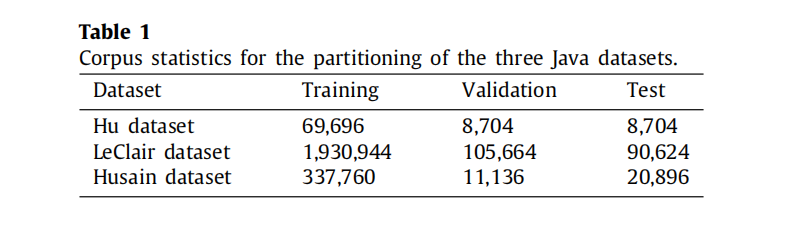
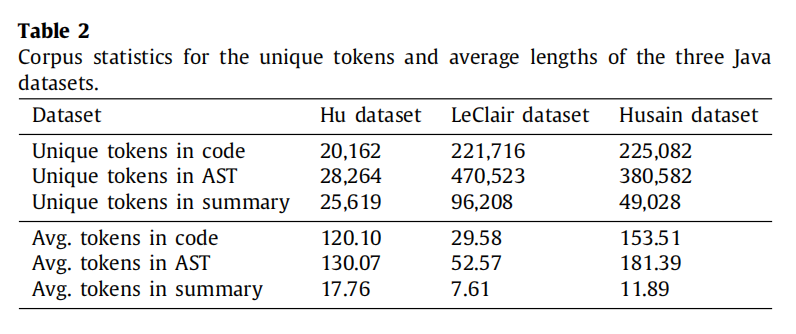
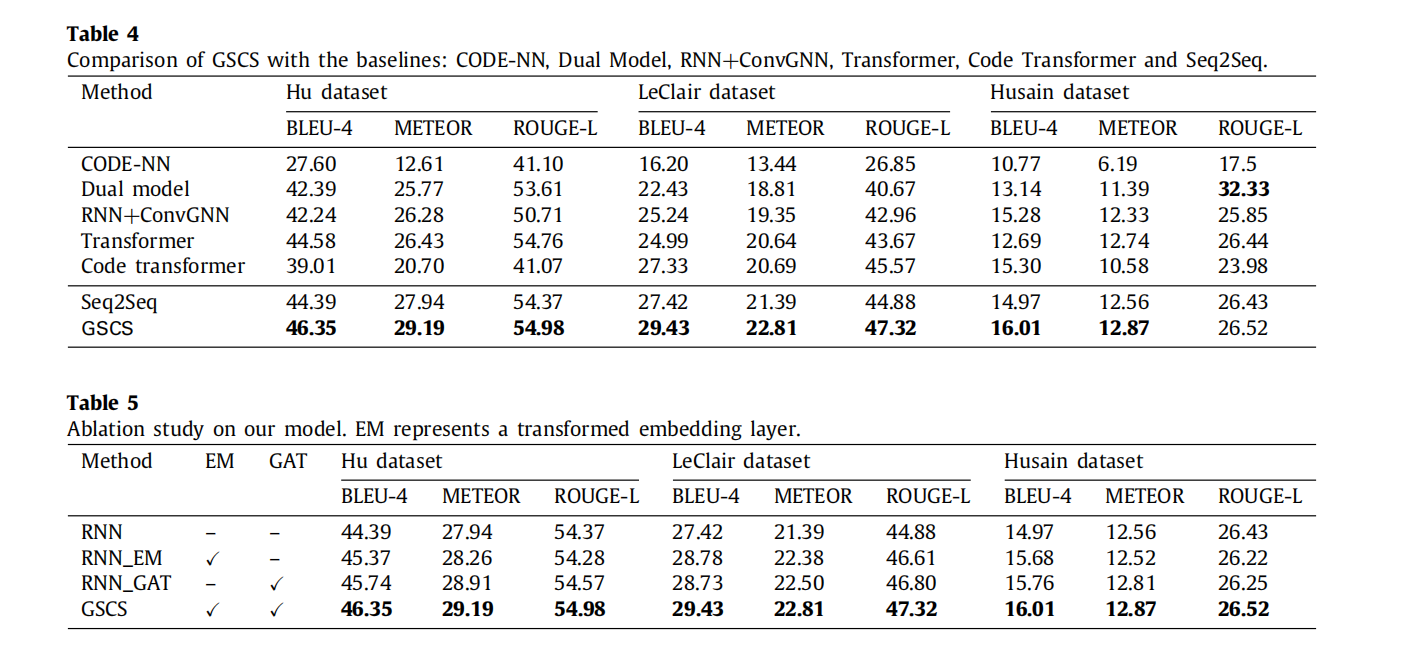