分解解码器为上下文网络和预训练模型
Abstract
在现有方法中还是很少,准确的摘要比如用没有出现在源文档的新短语来衡量依然很低。本文提出两种提高生成摘要的抽象程度的方法,第一种是将解码器分解为一个检索源文档相关信息的上下文网络,和一个预训练模型;第二种是一个新的指标
Introduction
第一个贡献通过将解码器分解为上下文网络和语言模型来降低解码器的提取和生成的responsibilities,上下文网络负责压缩源文档,语言模型负责生成简明释义
第二个贡献是一个混合目标,它联合优化与ground truth的n-gram重叠,同时鼓励abstraction
Model
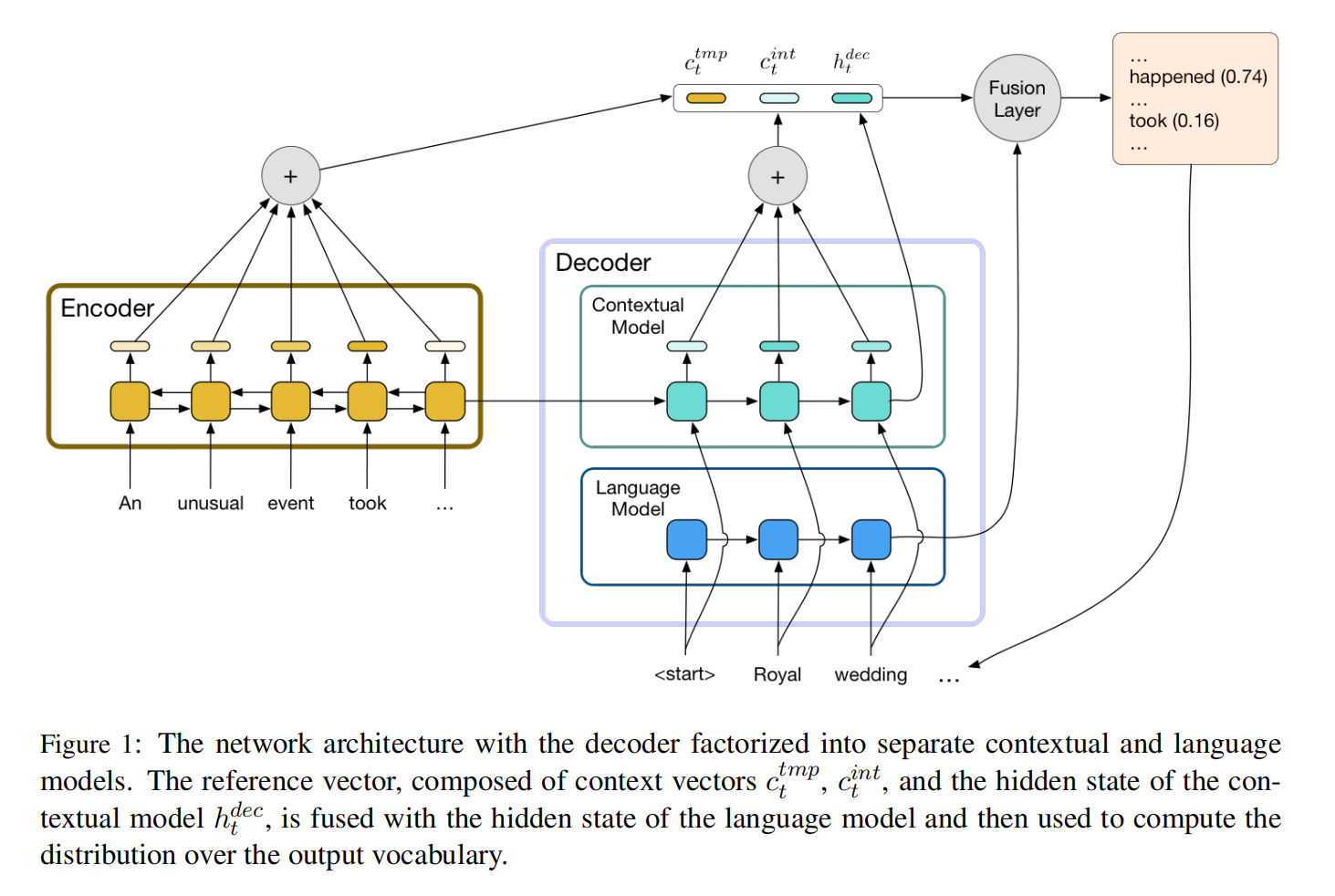
Base Model and Training Objective
编码器用的biLSTM

时间注意力上下文分数计算
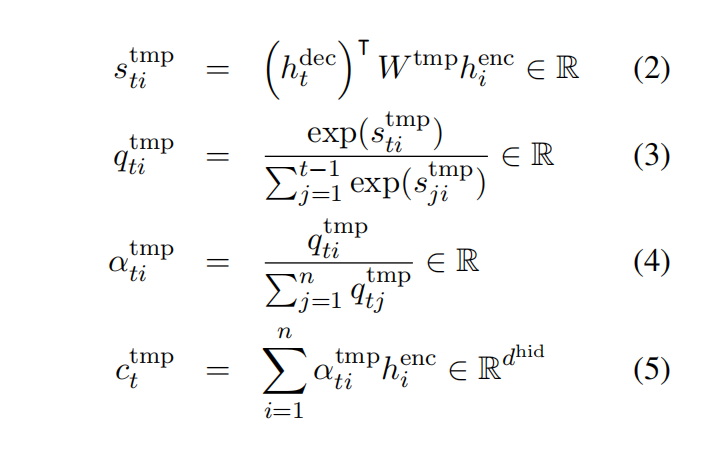
解码时内部注意力(intra-attention)上下文计算
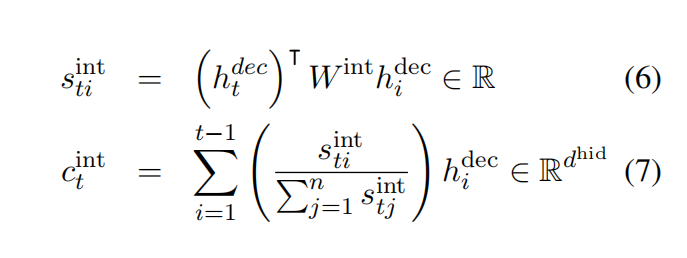
计算解码器从输出词表里生成的概率
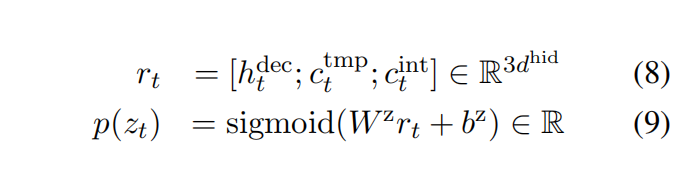
计算从固定词表选择词的概率

计算从原文档中复制的概率

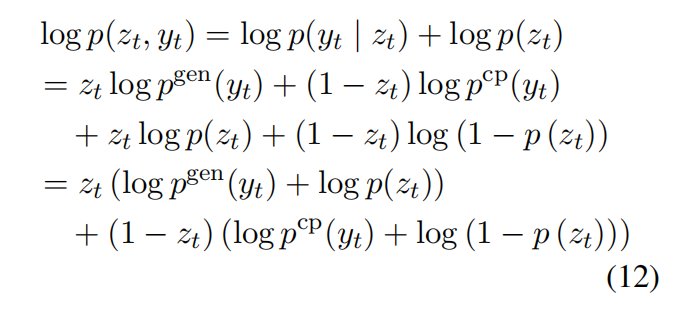
目标函数极大似然估计

策略学习使用ROUGE-L作为奖励函数,并使用贪婪解码策略作为self-critical baseline
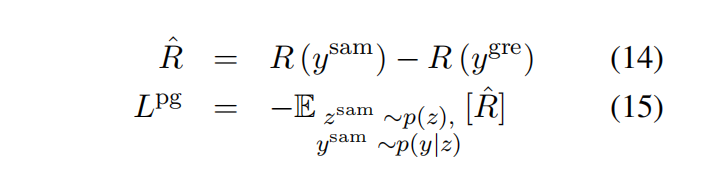
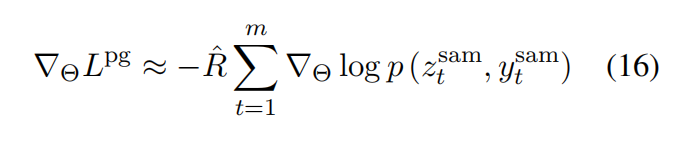
最终的损失是两者混合

Language Model Fusion
这种分解还有一个额外的好处,即通过在一个大规模的文本语料库上对语言模型进行预训练,可以很容易地整合关于流畅性或领域特定风格的外部知识
使用了3层无向LSTM,把最后一层语言模型的LSTM层的hidden state和公式8融合起来

$g_t$ 是门控函数
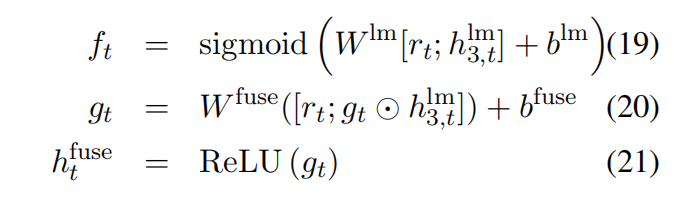
把公式10的输出分布替换为

Abstract Reward
看原文
Experiment
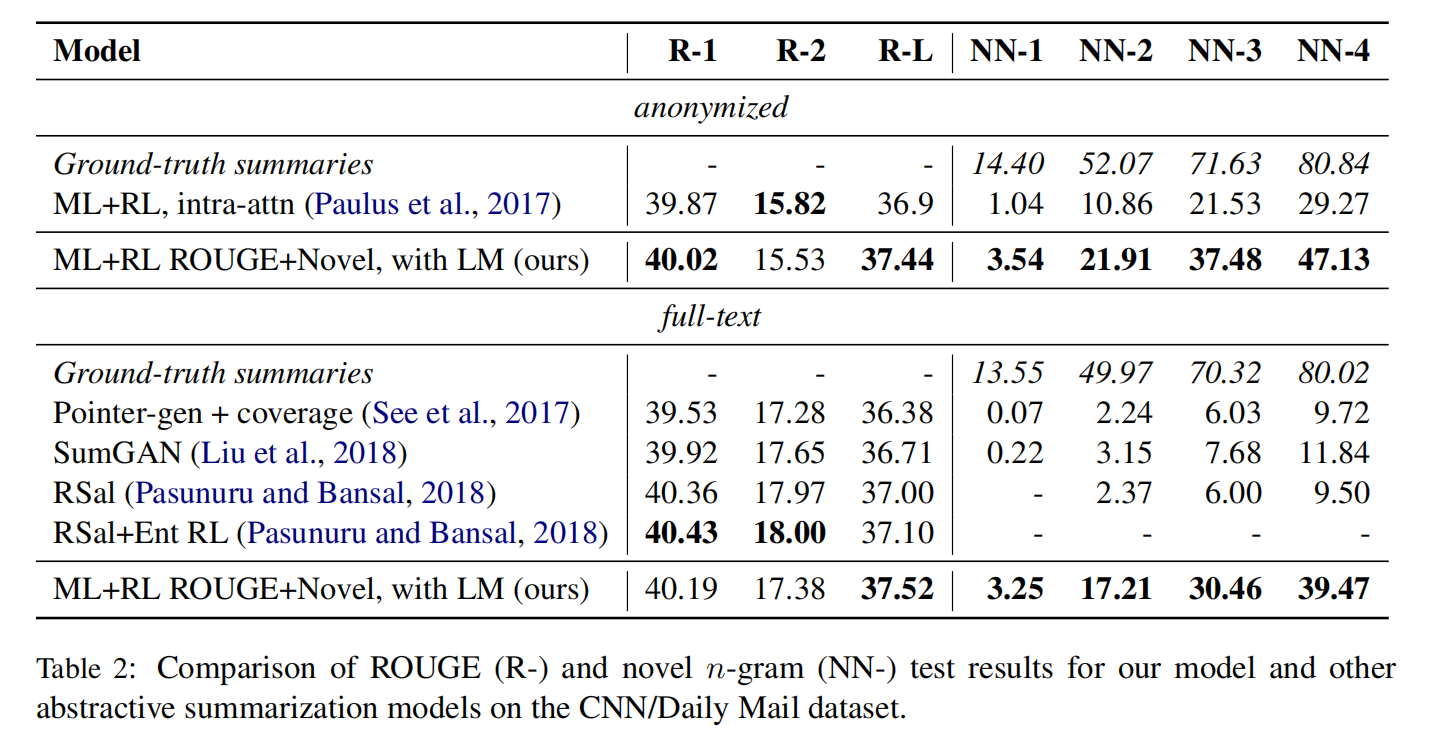
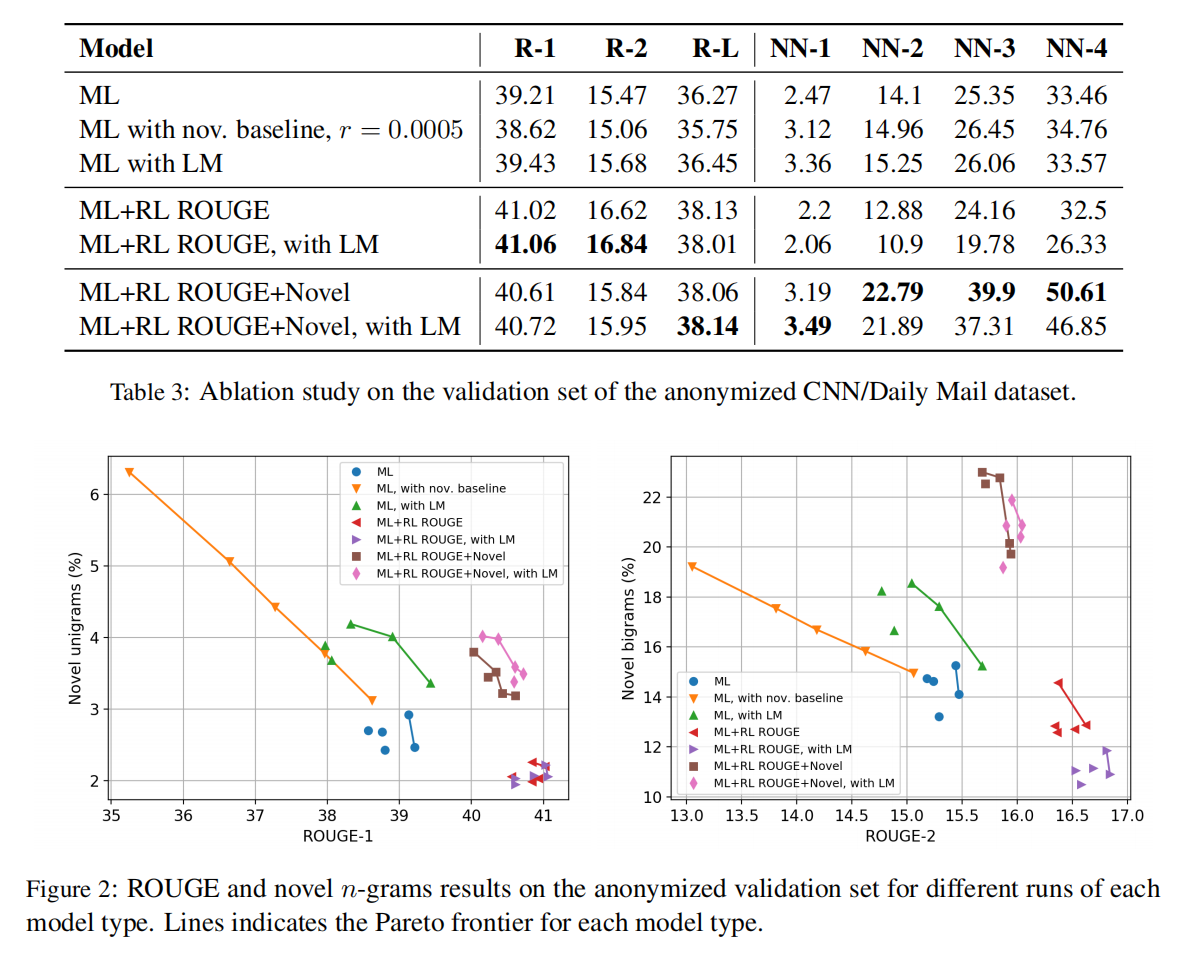