论文地址:When Geometric Deep Learning Meets Pretrained Protein Language Models
论文实现:https://github.com/smiles724/bottleneck
论文数据:The data of model quality assessment, protein-protein interface prediction, and ligand affinity prediction is available by https://www.atom3d.ai/. The data of protein-protein rigid-body docking can be downloaded directly from the official repository of Equidock https://github.com/octavian-ganea/equidock_public.
PLM-GNN:语言模型输入初始化GNN
Abstract
目前没有很先进的研究融合了蛋白质的不同模态来提升几何表征能力,本文把从预训练蛋白质语言模型的知识融合到多种SOTA的几何网络中,在多个任务中整体有近20%的提升,包括protein-protein interface prediction,model quality assessment,protein-protein rigid-body docking和binding affinity prediction
Introduction
相比于序列信息,用结构信息训练会少很多个数量级
通过自监督方法训练得到的PLM可以解锁编码在蛋白质序列中的信息,包括适应性,稳定性,功能以及自然序列分布
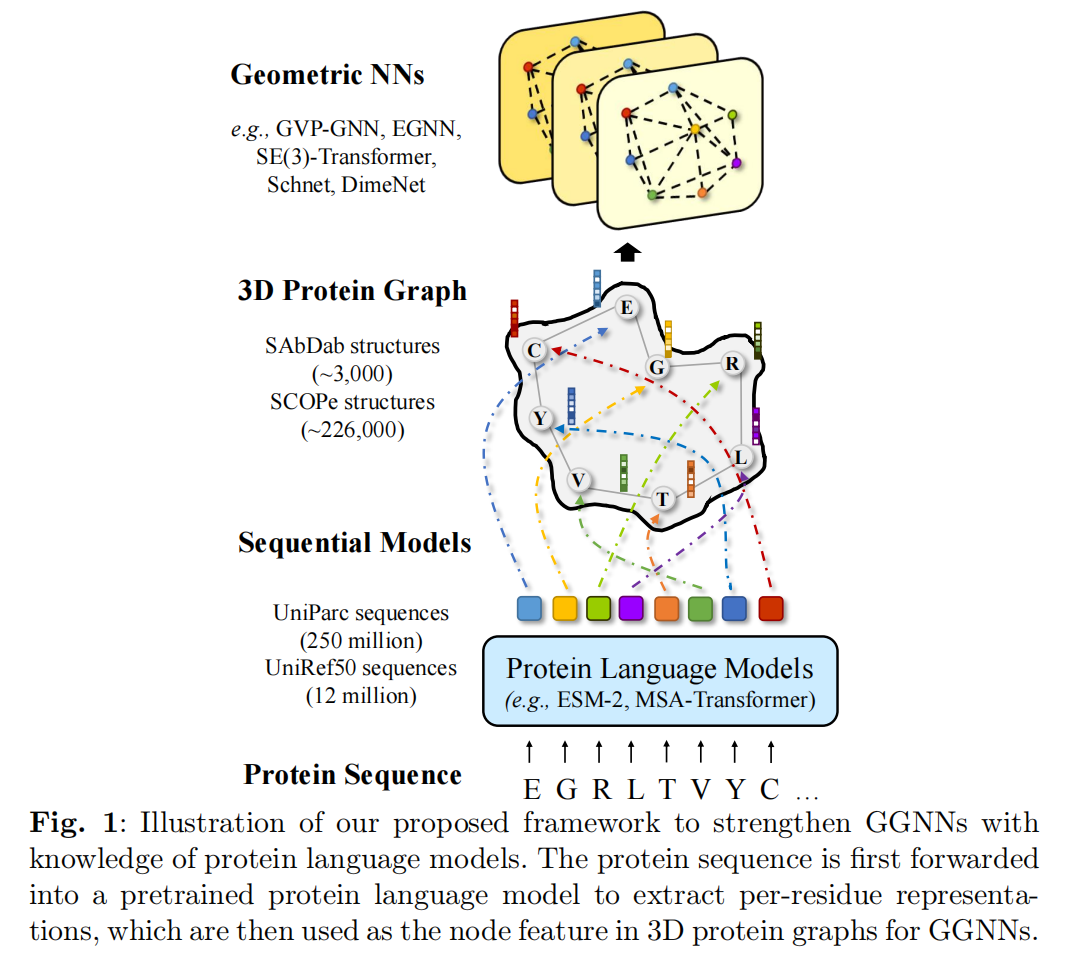
因此通过PLM提升GGNN的能力
Experiments
Task and Datasets
Model Quality Assessment (MQA)
旨在从一个蛋白质的一个大的候选结构池中选出最佳的结构模型。MQA方法通过预测一个候选结构和实验解决的结构的GDT-TS分数来评估,数据库是由过去18年来通过CASP提交的所有结构模型组成。MQA和protein structure ranking类似
Protein-protein Rigid-body Docking (PPRD)
从individual unbound structures预测protein-protein complex的3D结构,假设在蛋白质结合的时候没有构造变化,使用Docking Benchmark 5.5 (DB5.5)作为数据集
Protein-protein Interface (PPI)
当各自的蛋白质结合时两个氨基酸是否会接触,这是理解蛋白质相互作用很重要的问题,比如抗体蛋白识别抗原。使用Database of Interacting Protein Structures (DIPS)
Ligand Binding Affinity (LBA)
是对药物发现应用很关键的任务,预测与靶蛋白的一个候选药物分子交互长度,使用PDBbind database
Experimental Setup
使用pytorch和PyG,对MQA,PPI,LBA用GVP-GNN,EGNN和Molformer,对于PPRD用EquiDock作为backbone
Results
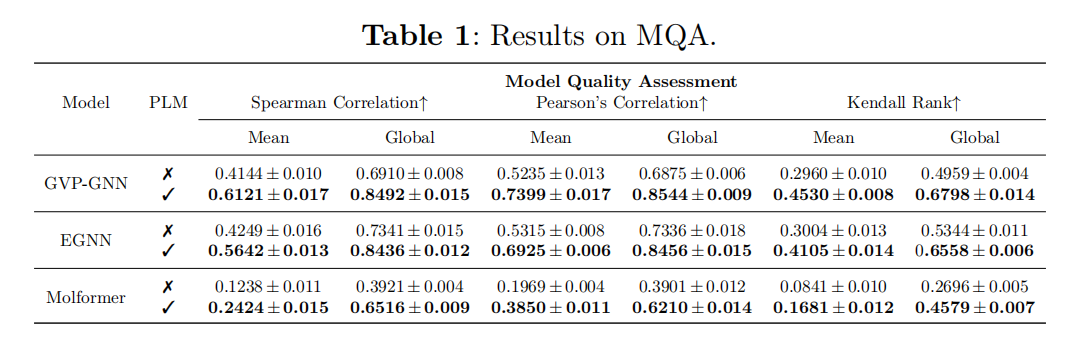
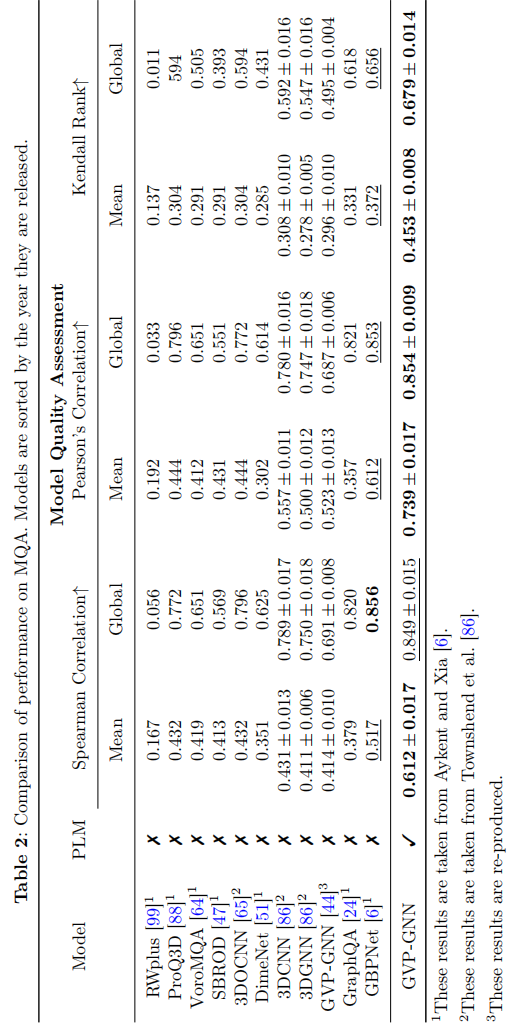
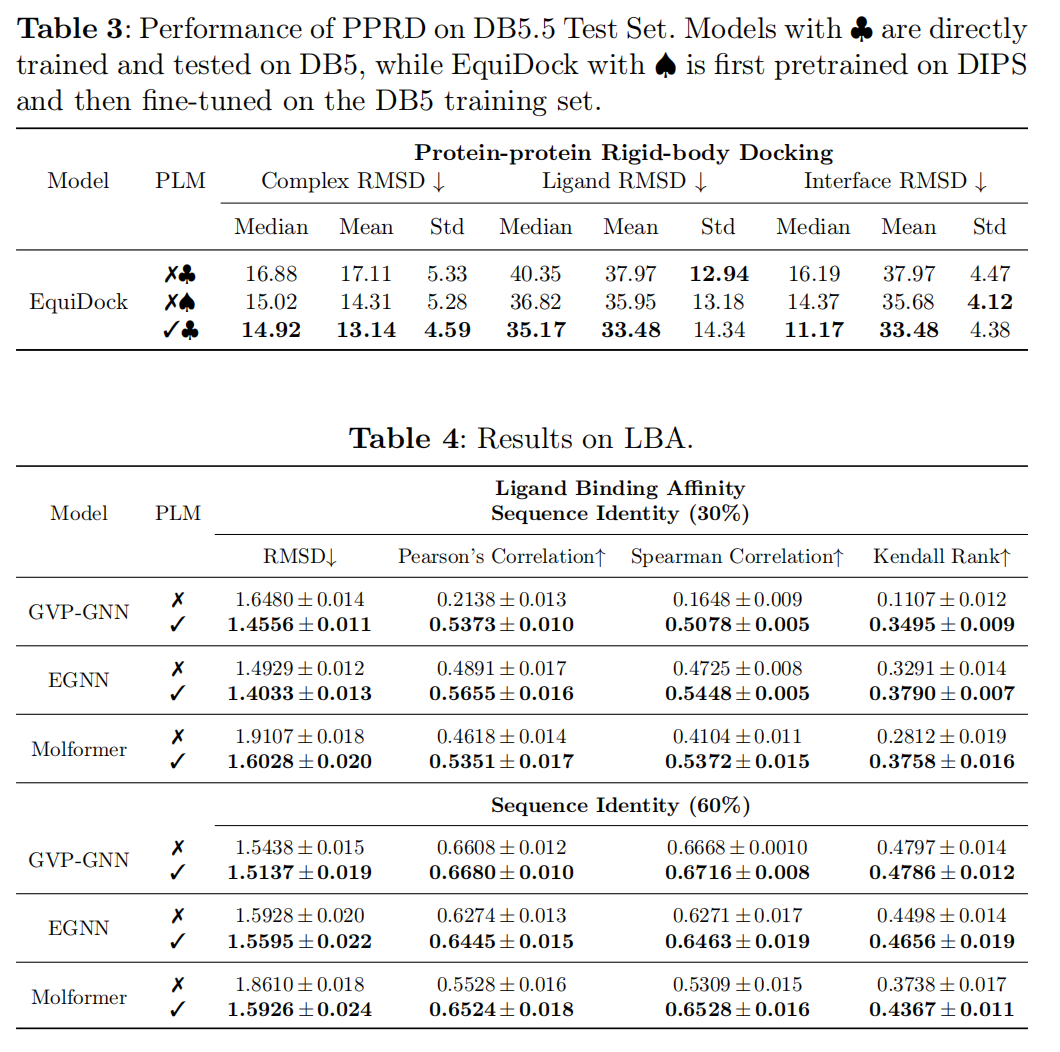
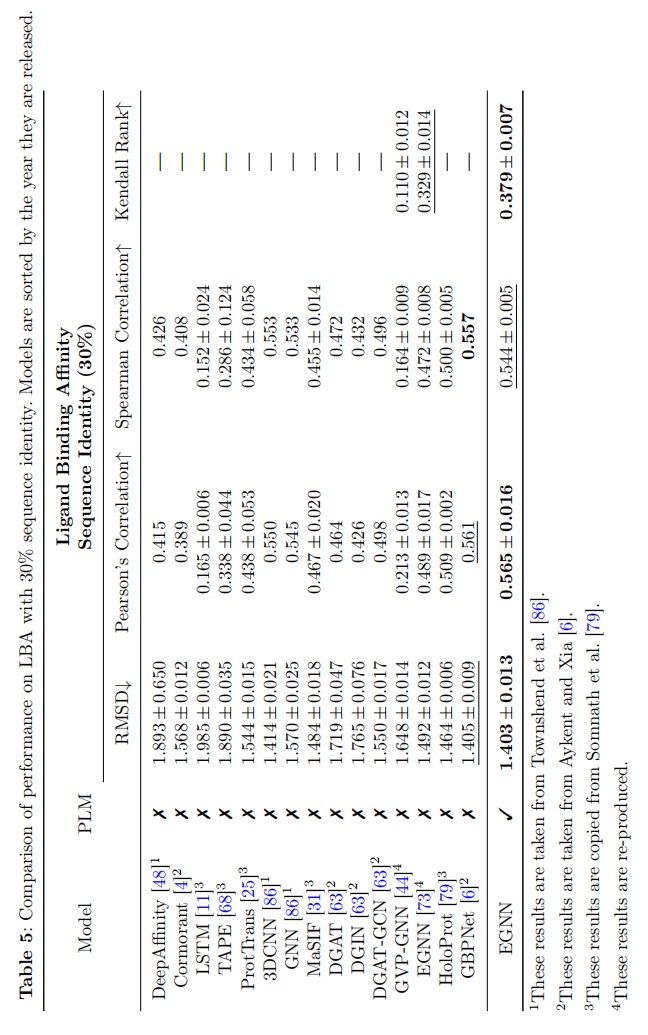
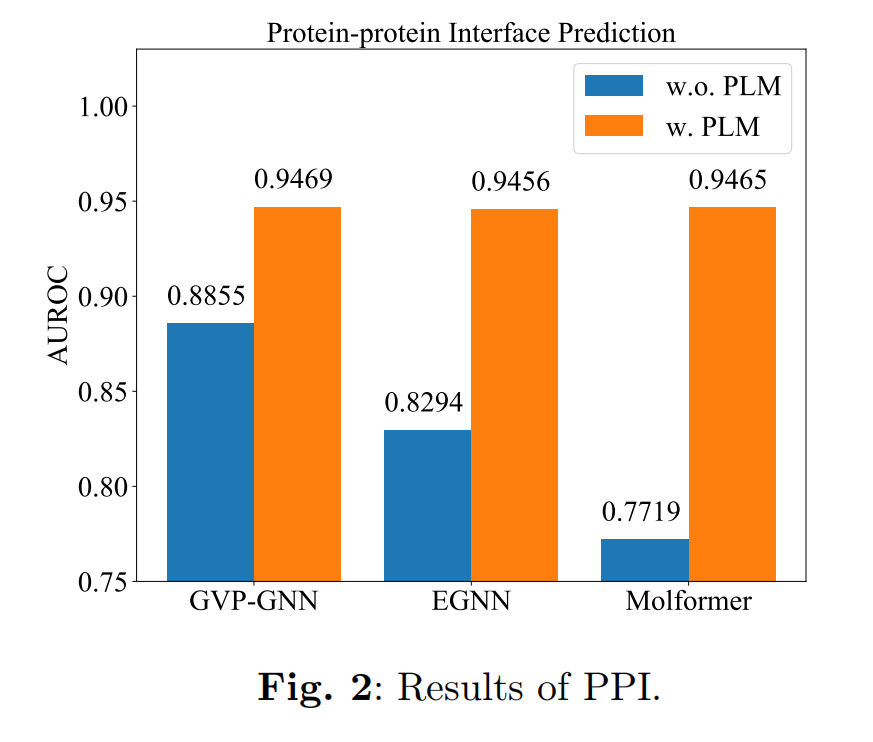
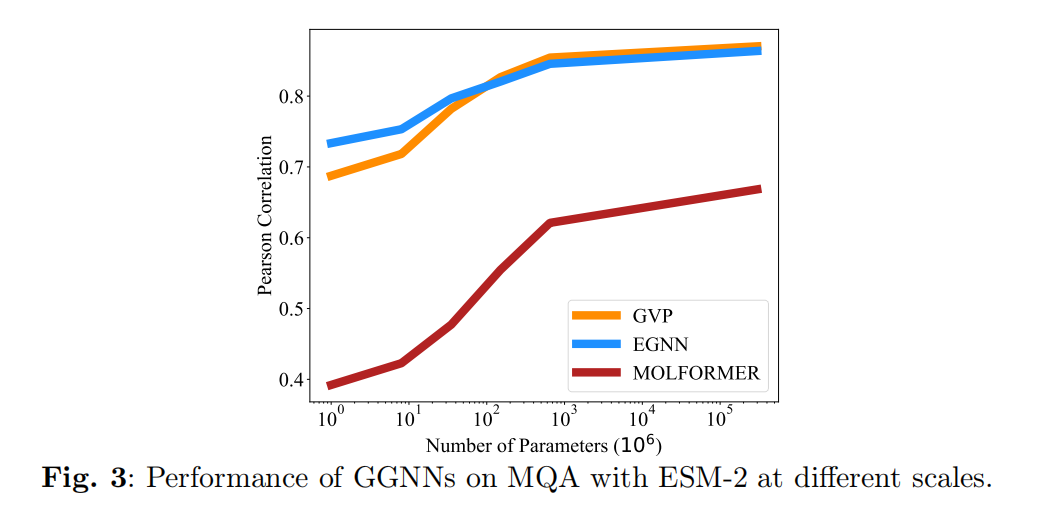
Conclusion
提出了一种简单有效的方法,即用现有的预训练蛋白质语言模型的氨基酸表征作为图网络初始化,在各个下游任务上有着明显的提升
Method
初始化的PLM是ESM-2,维度1280
现存的实验结构和原始氨基酸序列也存在不完整,有些残基的字符串因为现实因素缺失,通常的做法有直接使用片段信息来丢入模型forward,或是动态规划算法来实现成对序列对齐,并放弃PDB结构中不存在的残基
Sequence Recovery Analysis
GGNN在采用三维几何图形时的模式上主要是多样化的,输入特征包括距离、角度、扭转和其他阶数项。然而,在构建GGNN的三维图时,通常忽略了隐藏在蛋白质序列中的position index
Protein Graph Construction
三种形式来构建分子联通:r-ball graphs,fully-connected(FC),K-nearest neighbors(KNN),
Recovery from Graphs to Sequences
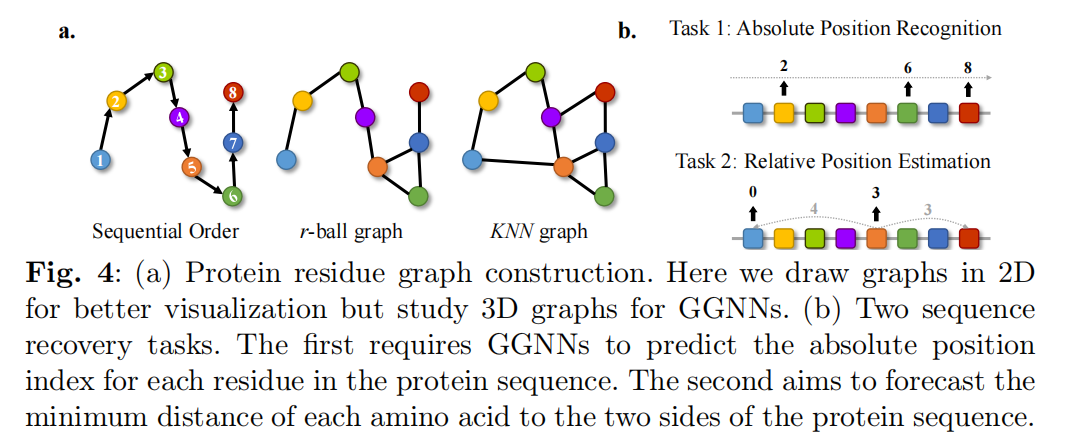
主要回答一个问题:现有的GGNN是否只从蛋白质的几何结构中就能识别序列位置顺序?
如图4所示,两个任务,预测绝对位置和预测相对位置
Results and Analysis
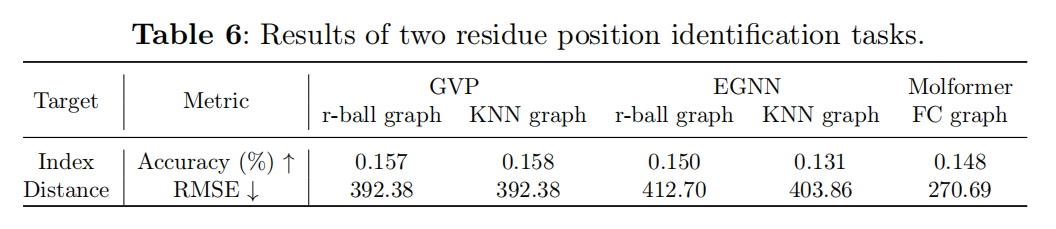
所有的GGNN都不能识别出相对位置和绝对位置信息,准确度低于1%,RMSE较高,主要是源于构造图的连通性的时候没有考虑到序列信息,更具体的说不像引文网络,社交网络,知识图谱,分子没有明确定义的边或邻接