论文地址:CoCa:Contrastive Captioners are Image-Text Foundation Models
Coca:text端用decoder同时增加数据
Abstract
本文提出了contrastive captioner (Coca),使用了contrastive loss和captioning loss
Apporach
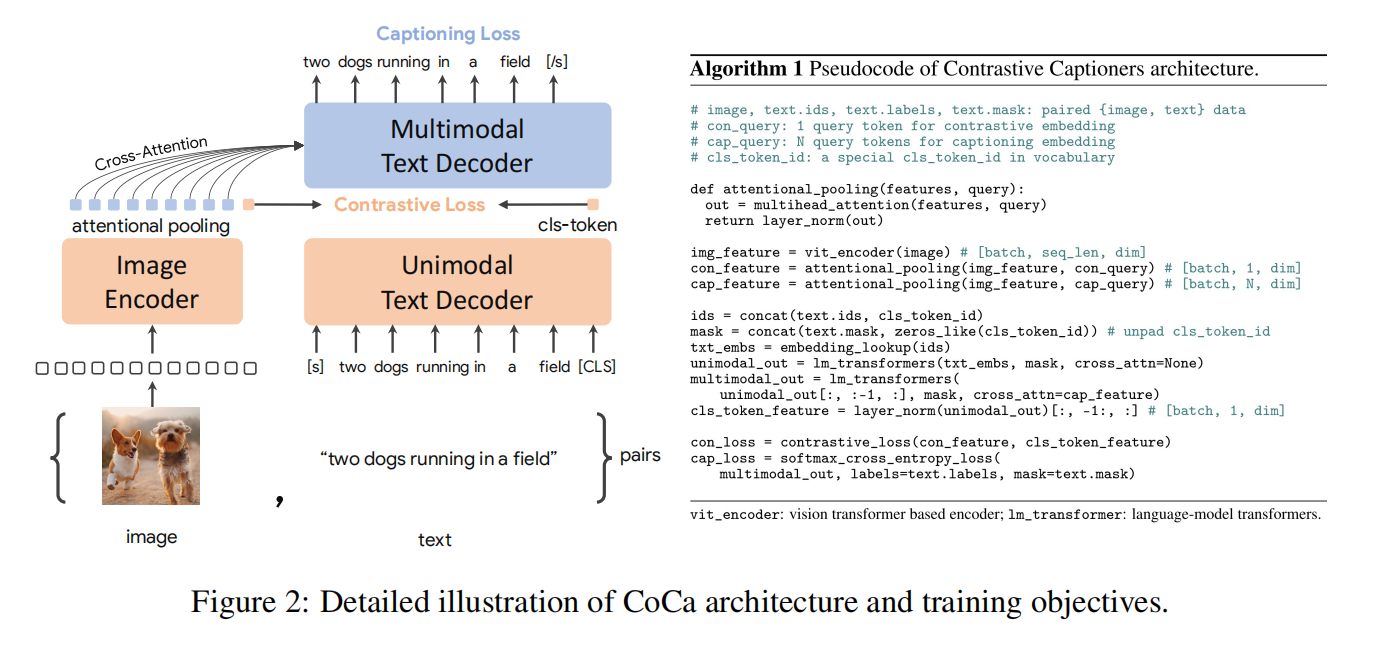
左边是imgae encoder,右边是text decoder拆分了两半,captioning loss就是language modeling loss。右边的text decoder从一开始就做的causal,所以只用forward一次,减少了计算量
并且模型预训练的数据非常大,有几十亿远超过去的scale,所以效果很好
Natural Language Supervision
Dual-Encoder Contrastive Learning

Encoder-Decoder Captioning

Contrastive Captioners Pretraining

Experiments
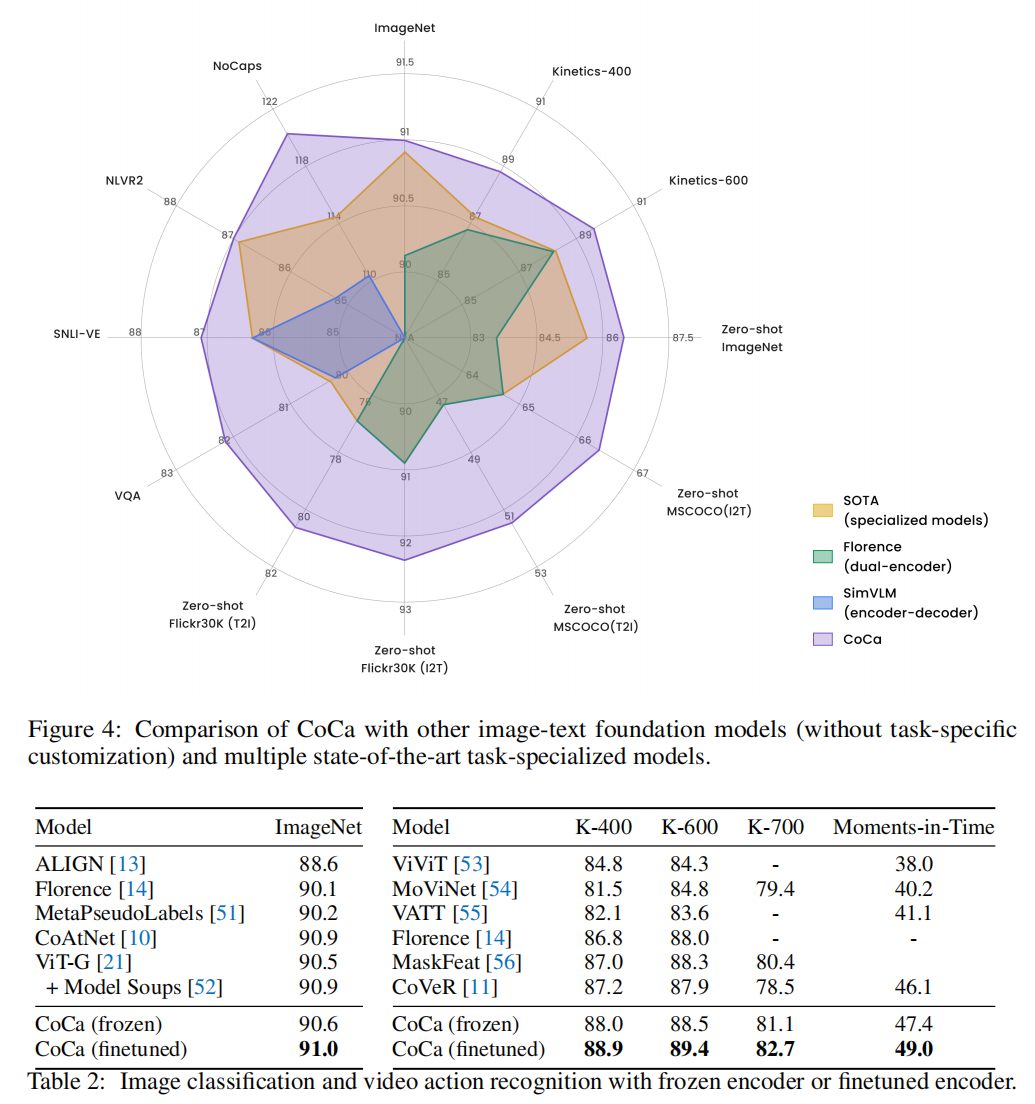
多边形的顶点是任务或数据集,同时单模态的工作也非常好