论文地址:Image as a Foreign Language:BEiT Pretraining for All Vision and Vision-Language Tasks
BEIT-3:多模态掩码语言模型
Abstract
提出了multi-way transformers,在图像上做掩码语言模型(Imglish),文本(English),图像文本对(parallel sentences)
Introduction: The Big Convergence
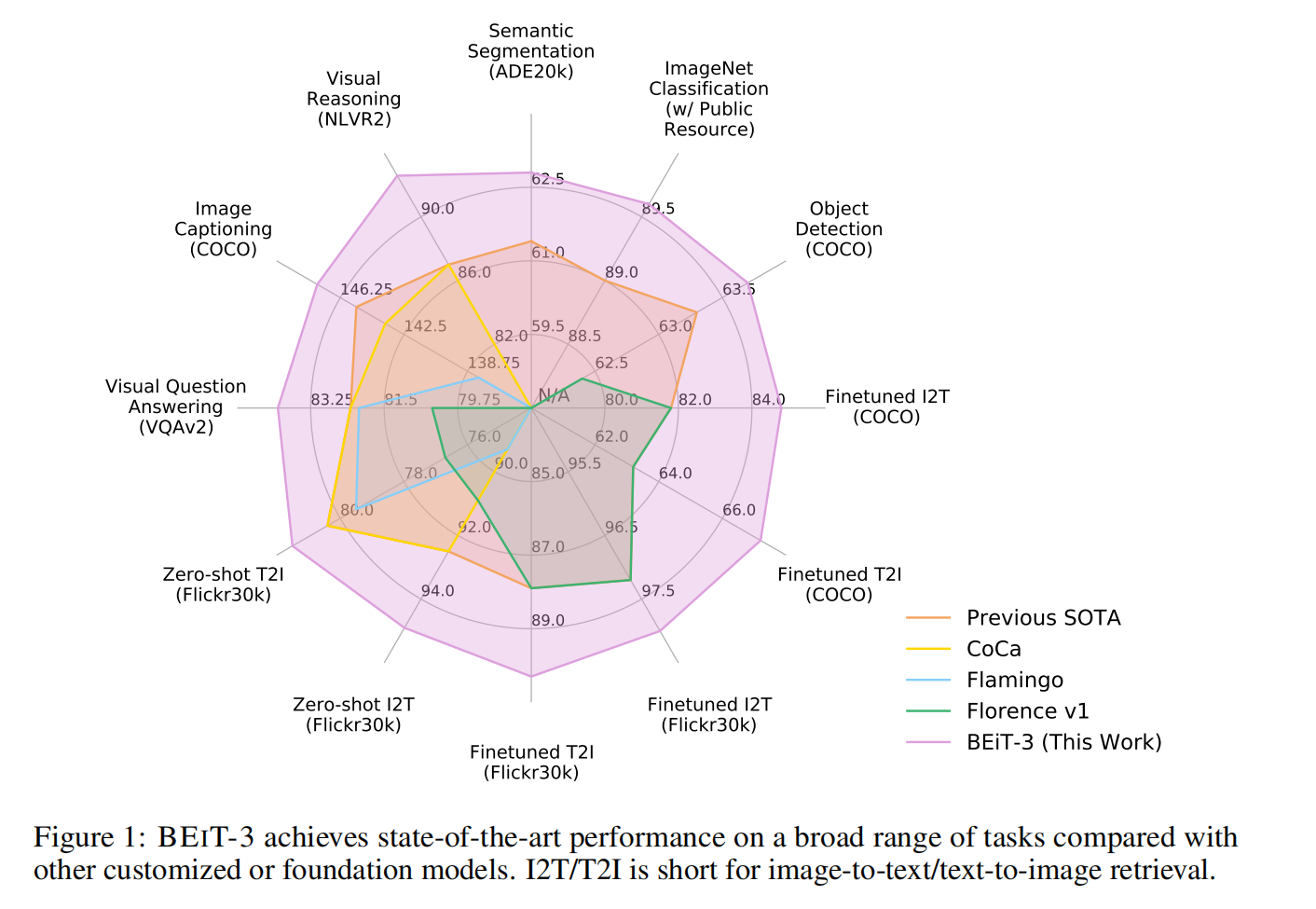
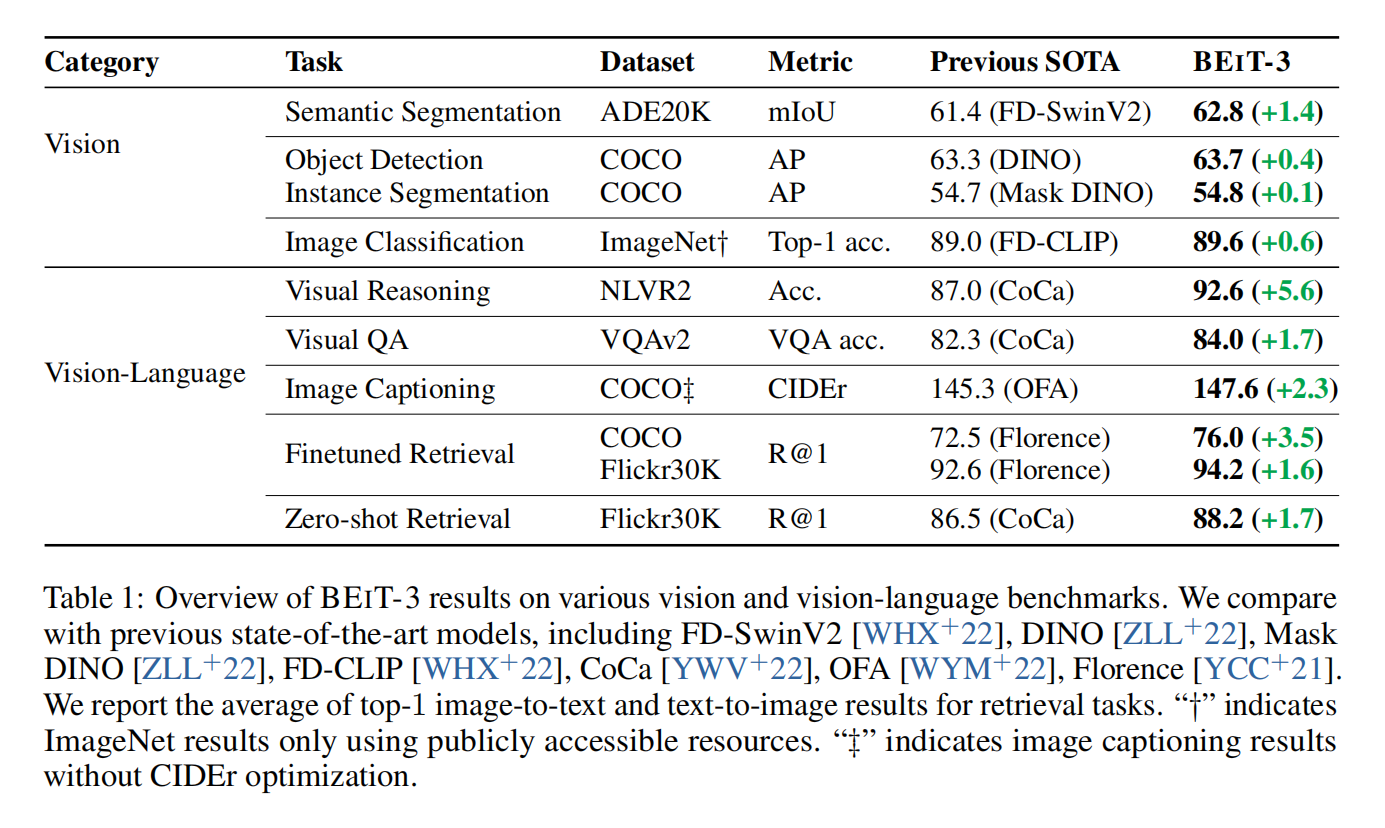
目前出现了语言,视觉和多模态的大一统趋势,这篇文章的主要目的也是把统一框架这种方法往前推进一步,这种融合趋势主要是以下三个方面:
- transformer从NLP转移到了CV和多模态,对于vision-language modeling而言,dual-encoder适合做快速retrieval,encoder-decoder网络适合生成任务,fusion-encoder架构很好的做image-text encoding。但是大部分的foundation model需要针对下游任务输入输出形式做一些改进
- mask modeling已经能够很好的应用到各个模态里,如果用更多的损失函数那么计算就不高效了,而且loss一多,weight怎么调也是一个大问题,有的Loss互补有的loss互斥,比较人工。所以只用了一个目标MLM,这样都可以视作sequence of tokens,图像和文本可以视作parallel sentences,句子1后跟句子2
- 如何提升模型和数据大小,只有一个capacity很大的模型才能handle更多的任务,所以模型参数扩展到了billion级别,但数据还是使用的public resources
BEIT-3: A General-Purpose Multimodal Foundation Model
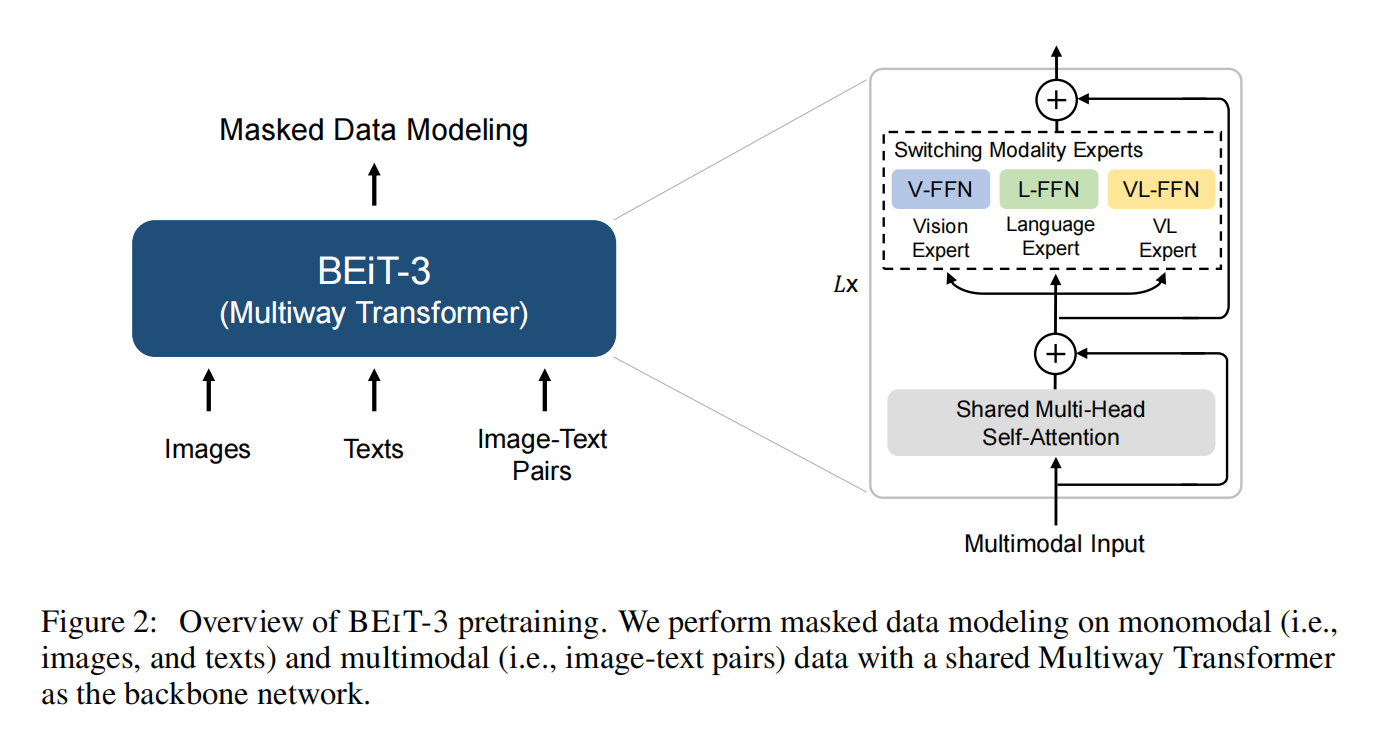
模型本身就是VLMO,前面的multi-head self-attention是共享权重的,后面的FFN是根据不同的模态进行训练的。有可能是遮住图像,有可能是遮住文本,总之都是完形填空
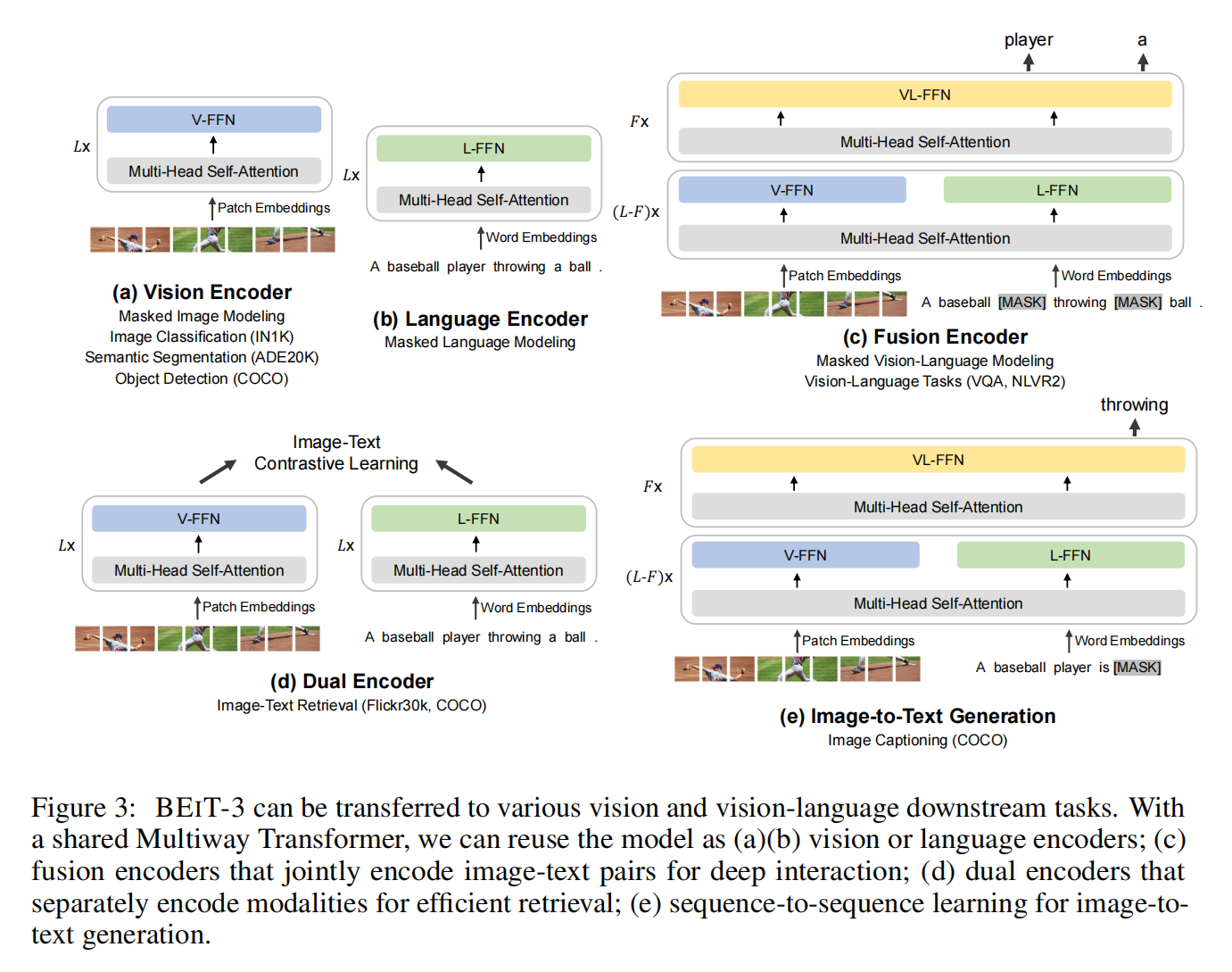
转移到下游任务
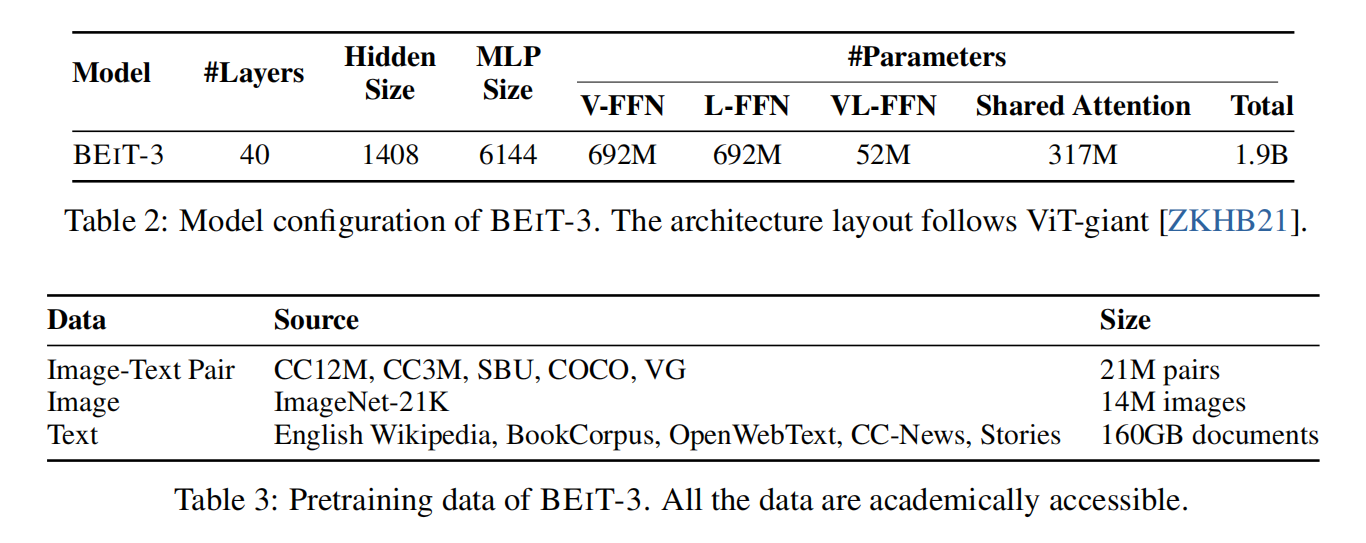
Experiments
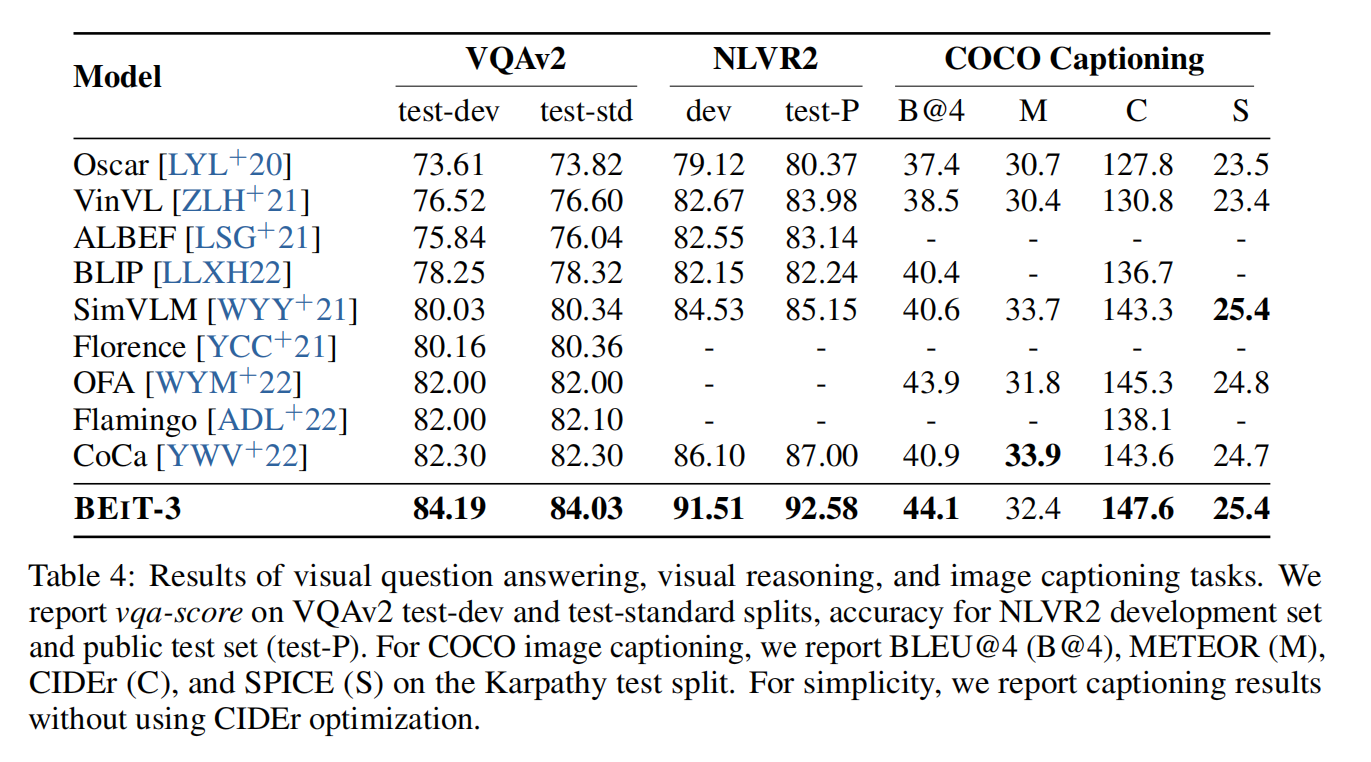
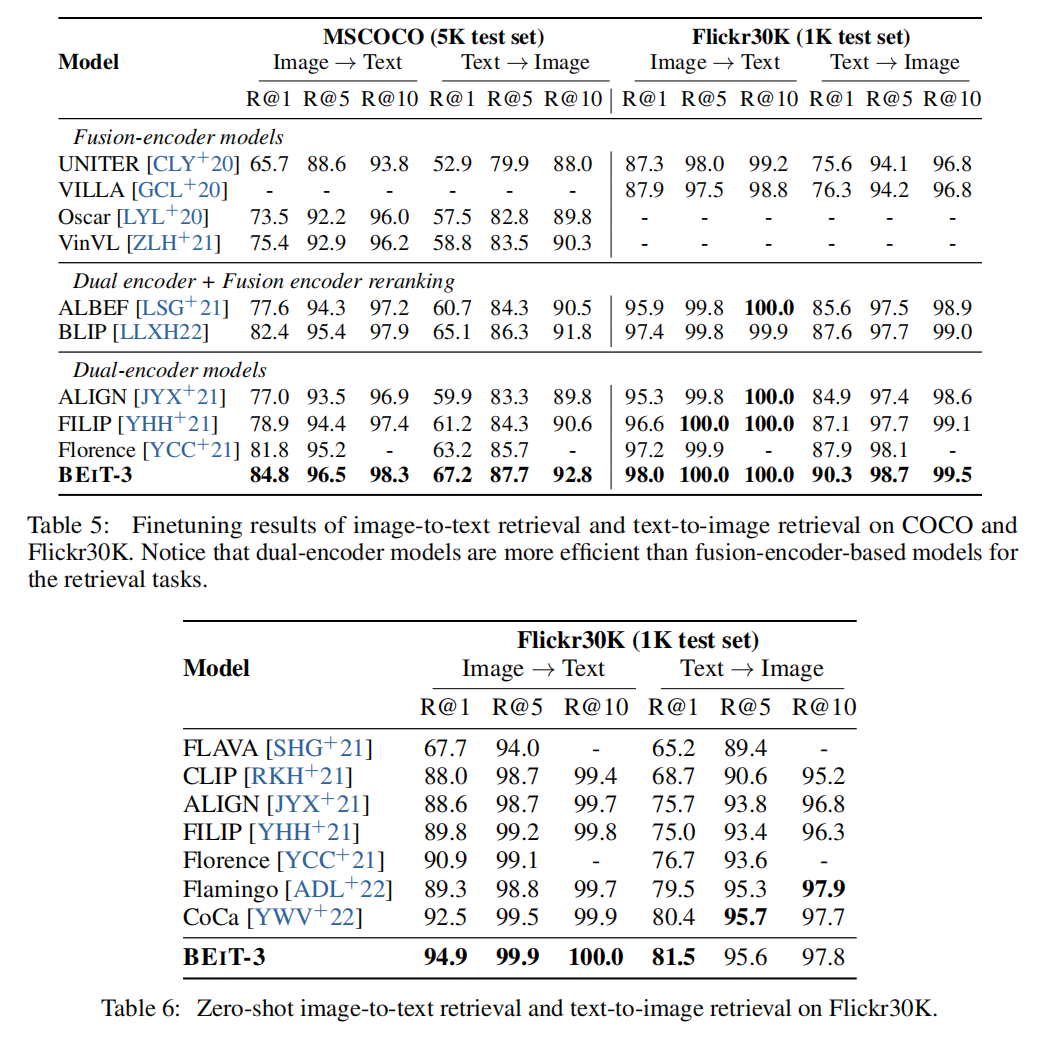
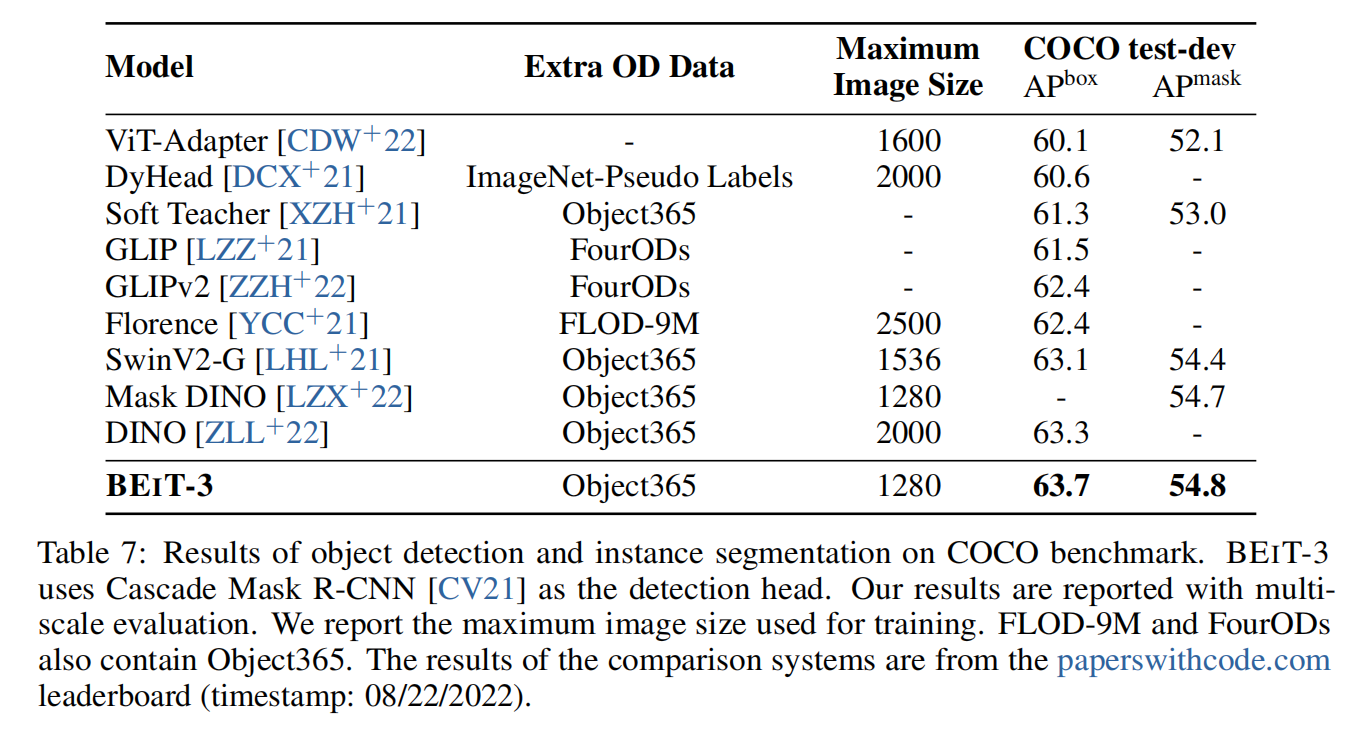
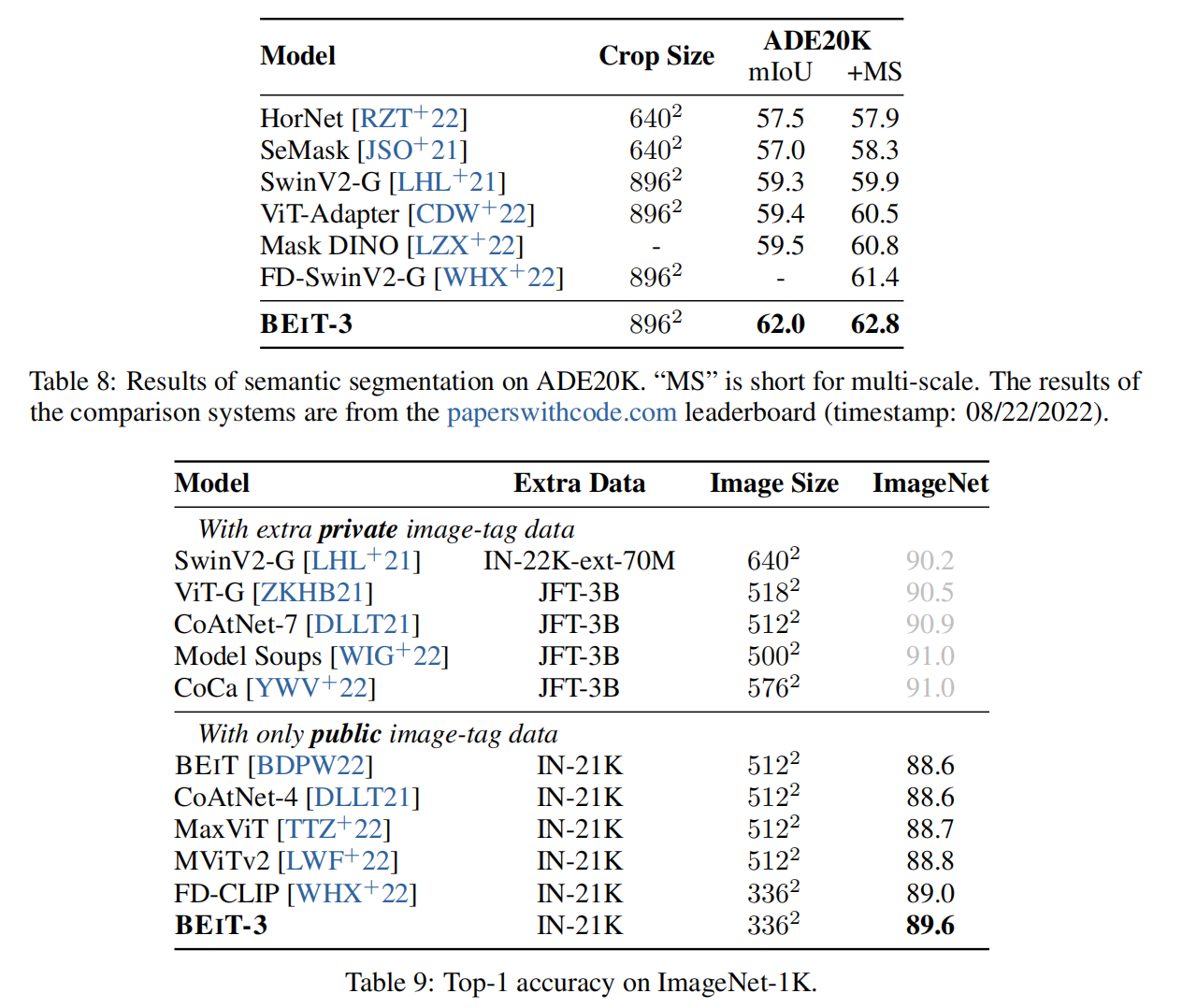