论文地址:Enhancing Protein Language Models with Structure-based Encoder and Pre-training
ESM-GearNet: 多视图对比学习ESM-1b+GearNet
Abstract
预训练语言模型能够建模氨基酸之间的内在联系,加上结构信息之后增强PLM的能力,本文探究了不同的结合结构编码器和PLM编码器的组合,提出了ESM-GearNet,使用对比学习预训练,蛋白质结构和其子序列结构对齐
Introduction
通过掩码语言建模损失进行预先训练,现有的PLM可以很好地捕获共同进化信息和隐式捕获残留间的接触信息。然而,由于它们没有明确地将蛋白质结构作为输入,因此它们是否能够捕获详细的蛋白质结构特征是值得怀疑的
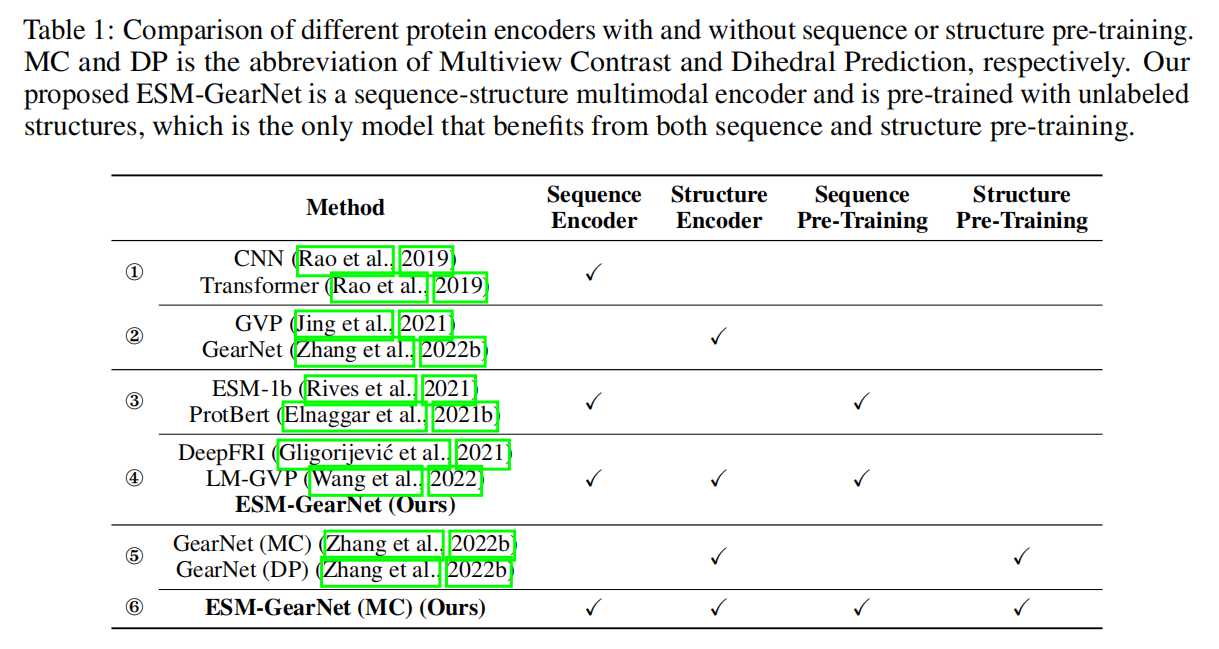
Methods
Enhanceing Protein Language Model with Protein Structures
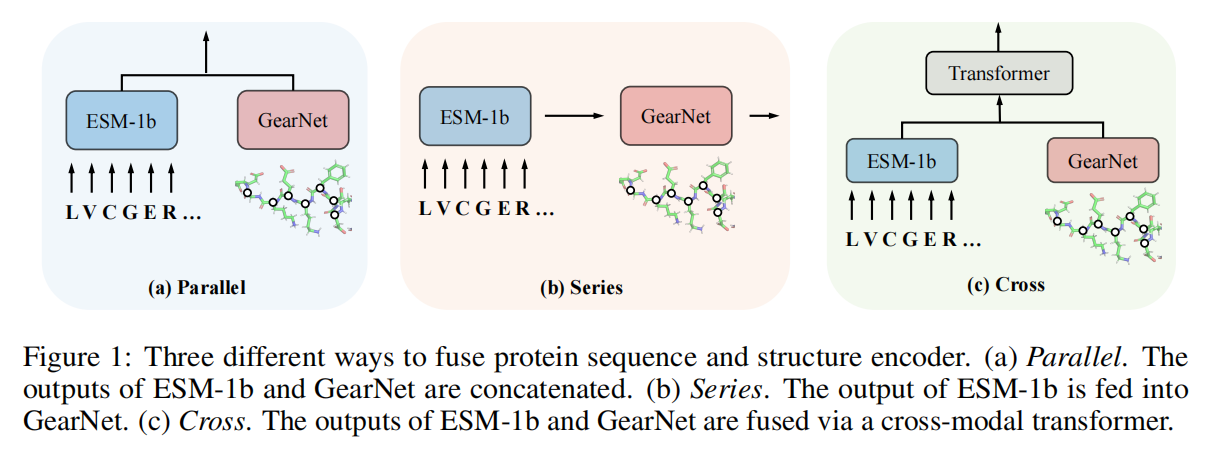
为了避免大幅改变模型表征,使用了比ESM-1b和GearNet更小的学习率
Pre-training ESM-GearNet with Unlabeled Protein Structures
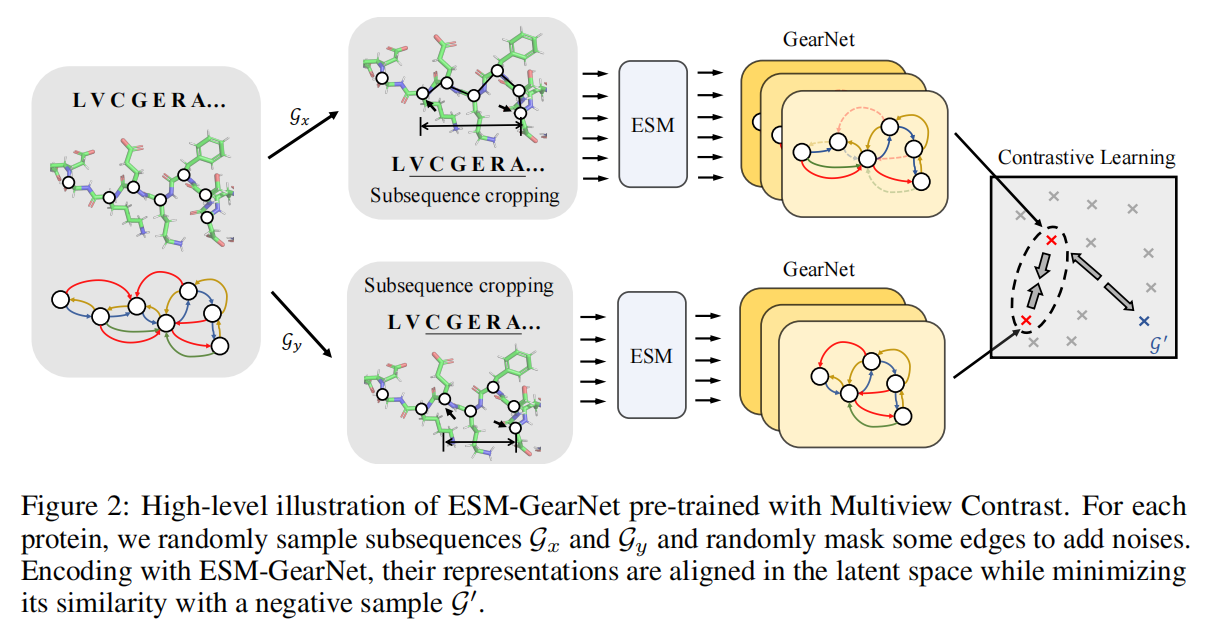
Experiments
Experimental Setup
Pre-training dataset
AF数据库的v1和v2,365K个蛋白质组预测和440K的swiss-prot预测
Downstream datasets
Enzyme Commission (EC) number prediction
Gene Ontology (GO) term prediction molecular function (MF)包括biological process (BP) and cellular component (CC)
Training
50 epochs on AlphaFold Database and for 200 epochs on EC and GO prediction
多视图对比学习裁剪的子序列长为50,mask ratio为15%,InfoNCE loss的temperature为0.07,batch size为256,学习率2e-4
下游任务batch size为2,学习率1e-4,ReduceLROnPlateau scheduler with factor 0.6 and patience 5
Results
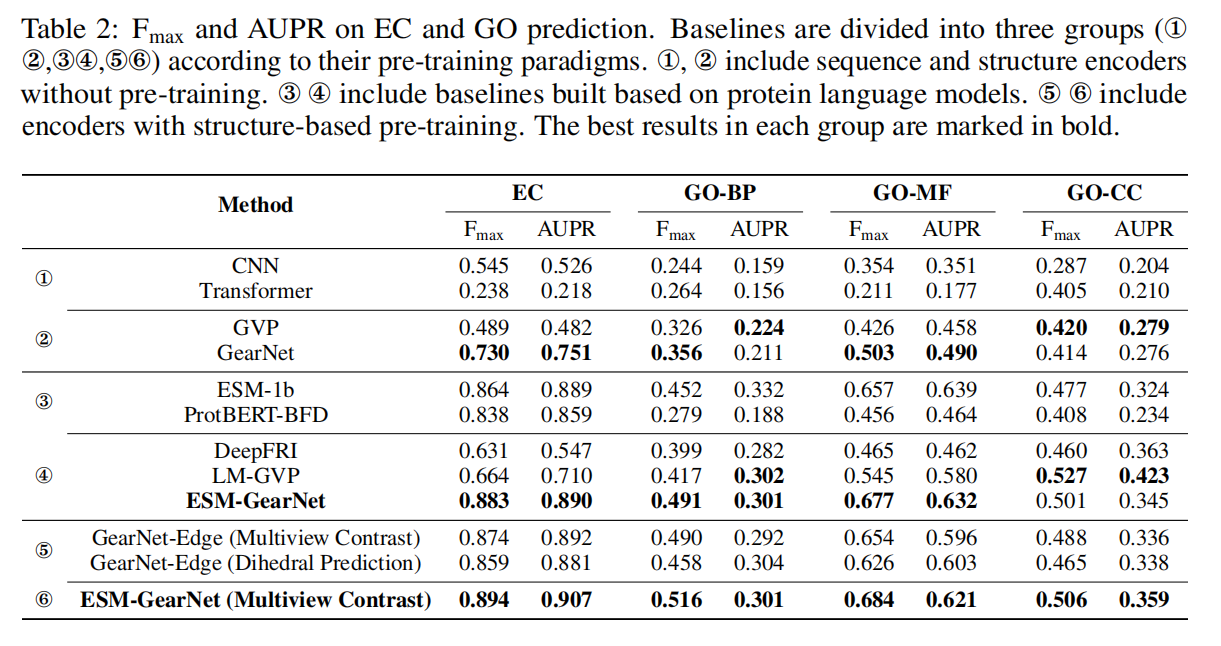
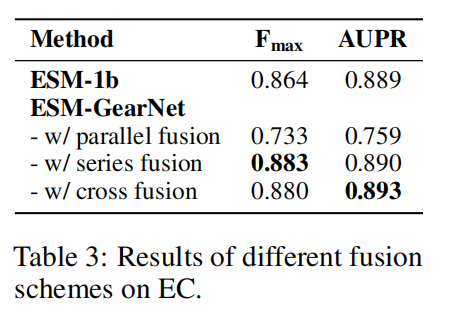