论文地址:Light attention predicts protein location from the language of life
LA-ProtT5: 语言模型表征+attention预测蛋白质定位
Abstract
知道蛋白质在细胞中的作用对于表征生物过程很重要,但目前大多数已知的蛋白质是没有的,目前专家设计的需要MSA信息或机器学习预测,搜索MSA和专家设计是比较昂贵的。这里使用来自蛋白质语言模型的嵌入在没有MSA的情况下进行蛋白质定位预测,取得了SOTA
Introduction
Prediction bridges gap between proteins with and without location annotations
在分子生物学中标准的预测工具是homology-based inference (HBI),从已经注释的相似蛋白迁移到未注释的蛋白上,但是HBI方法在大多数蛋白上不可靠或者不可用
机器学习方法精度低,但是在大多蛋白上是可用的,比如使用MSA做输入,但随着数据库的增长,MSA的开销变得巨大
Protein language models better represent sequences
使用蛋白质语言模型的表征来预测蛋白质定位,不需要MSA,使用一种Light Attention (LA)
Methods
Data
标准的DeepLoc和从swissprot中构建的新的setHARD
Models
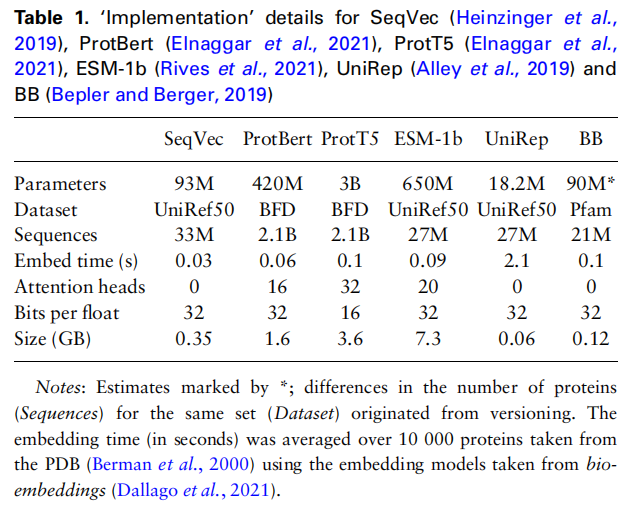
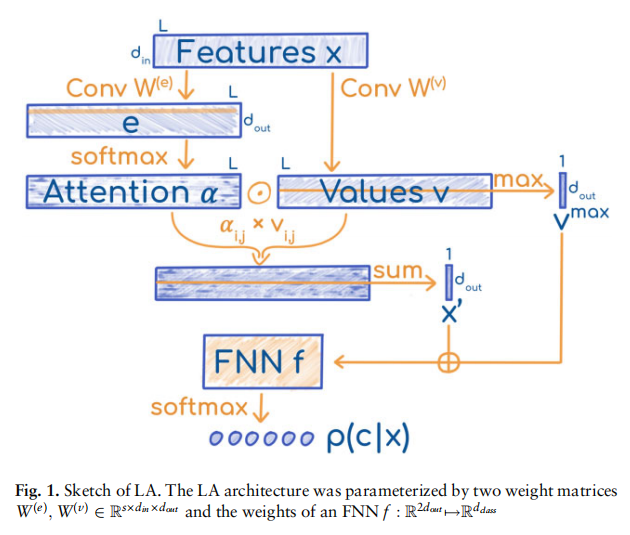
Results
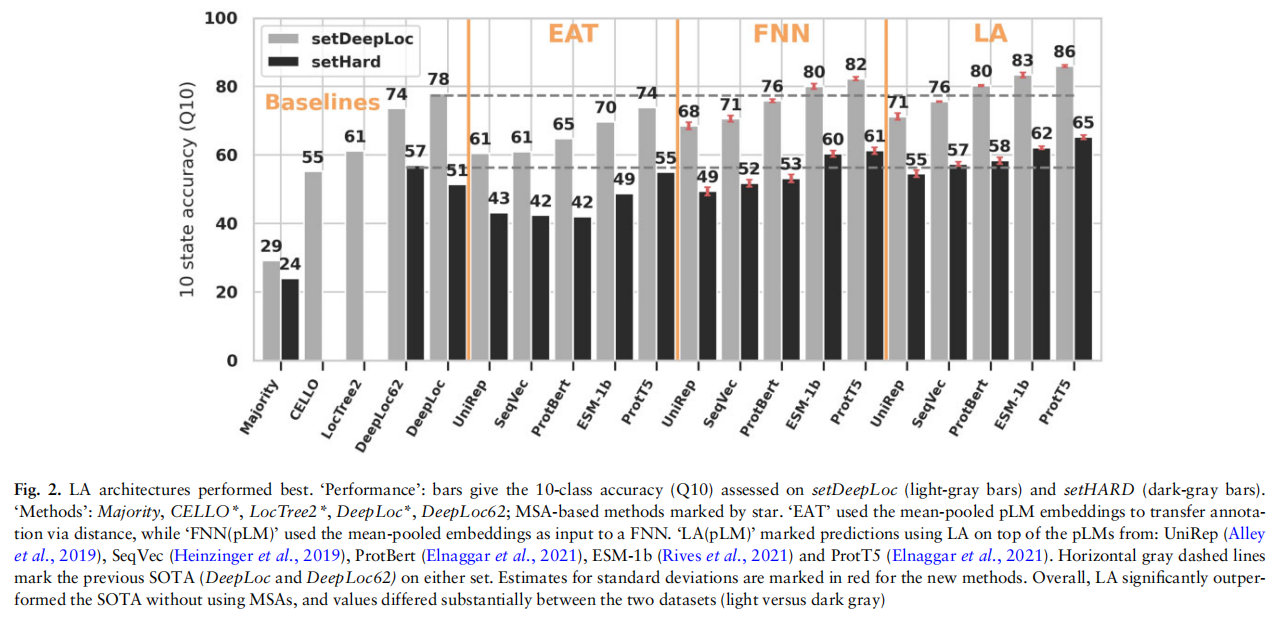
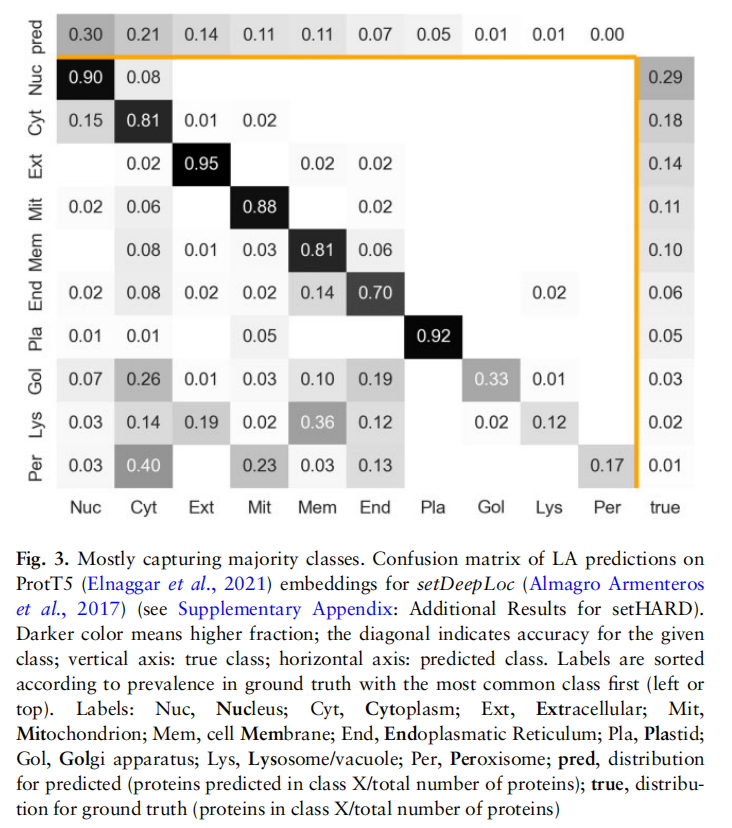
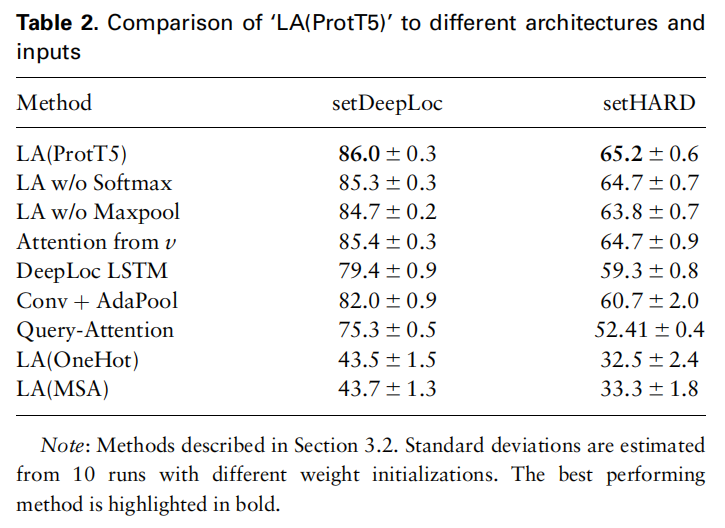
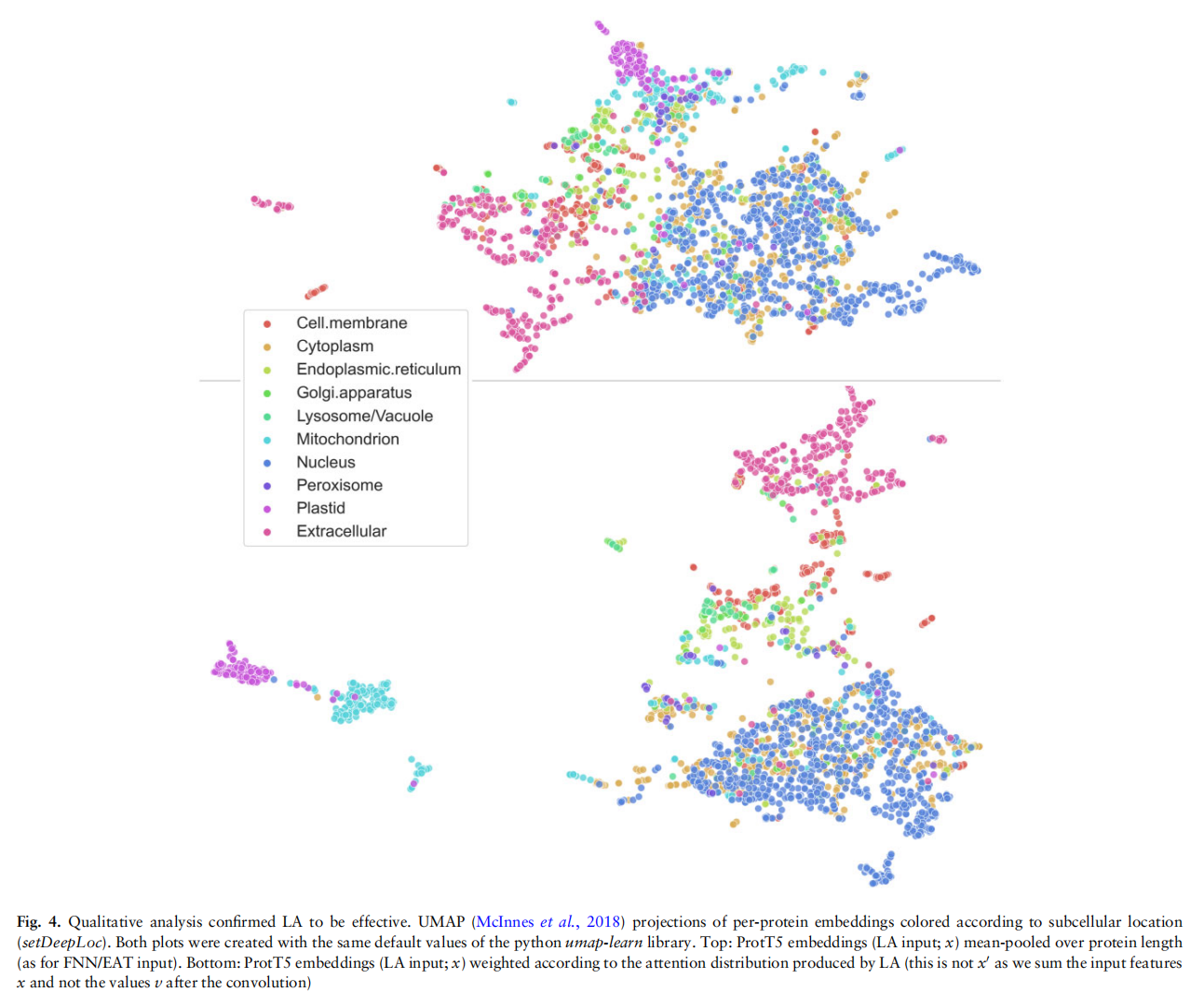