论文地址:ProteinGym: Large-Scale Benchmarks for Protein Design and Fitness Prediction
ProteinGym v1:蛋白质fitness benchmark
Abstract
提出了ProteinGym v1.0的fitness benchmark。同时设计了一个完整的evaluation framework,评估了超过40个模型
Introduction
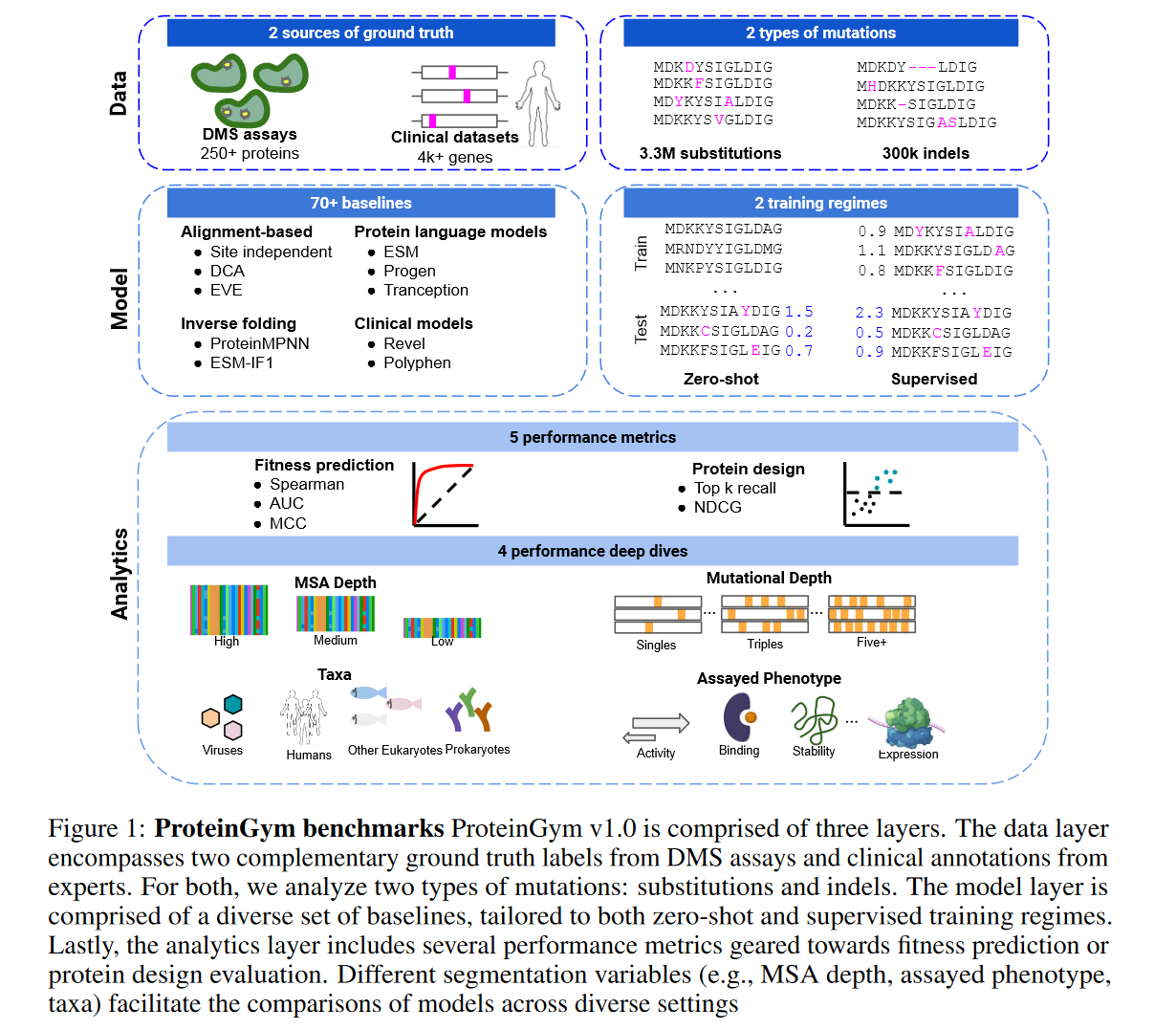
学习蛋白质序列或结构到最终性质的预测称为fitness landscape,而这些benchmark制作的越准确就越能帮助评估机器学习方法的有效性。收集了超过250个DMS合计3.5M个突变序列,囊括超过200个protein famliy
ProteinGym benchmarks
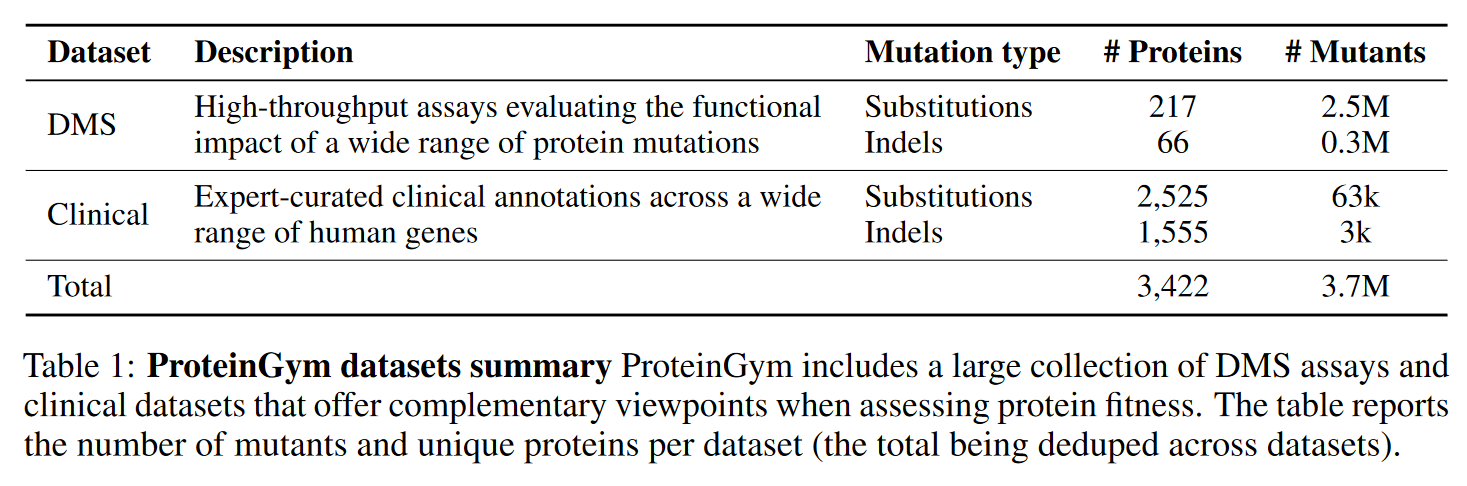
Evaluation framework
Zero-shot benchmark
DMS assays
因为蛋白质功能和fitness的非线性,spearman’s rank correlation coefficient是有效评估的
同时有的DMS存在双峰情况,相关系数不一定是一个好的选择,所以还使用Area Under the ROC Curve (AUC) 和Matthews Coreelation Coefficient (MCC),使用binarized experimental measurements
更重要的是模型需要捕获性质更强的蛋白质而不是数据的分布,还使用了Normalized Discounted Cumulative Gains (NDCG)
同时还计算了Top K Recall,K是DMS的前10%
Clinical datasets
计算AUC和precision-recall curves
Supervised benchmark
DMS assays
使用了三种不同的交叉验证方案:
- Random scheme:每个突变是随机分配的五折中的一块
- Contiguous scheme:把序列拆分五块
- Modulo scheme:使用模算子为每个折叠分配位置,以获得总共5次折叠,position number和折数的模
评估Spearman’s coreelation和Mean Squared Error (MSE)
Results
Substitution benchmarks
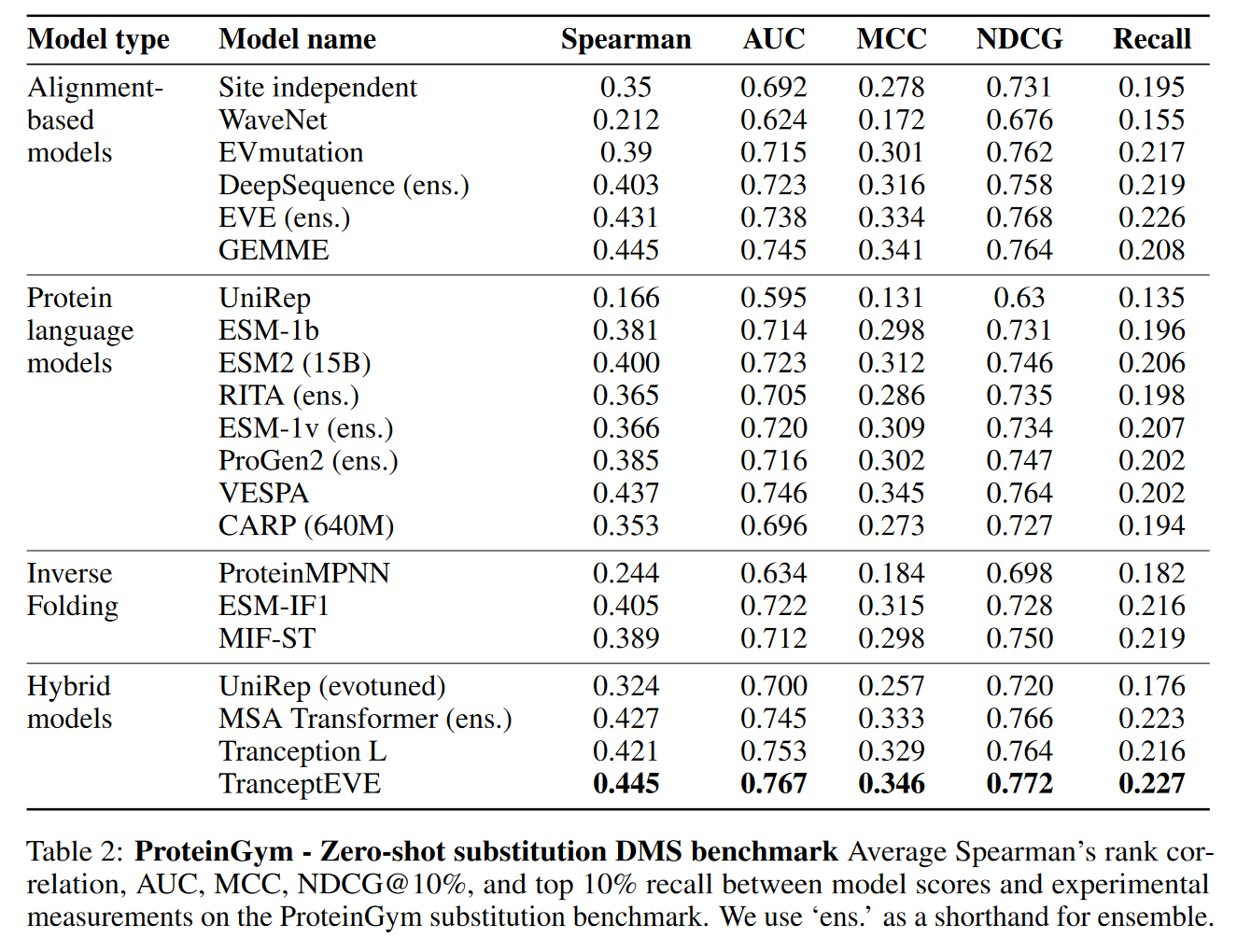
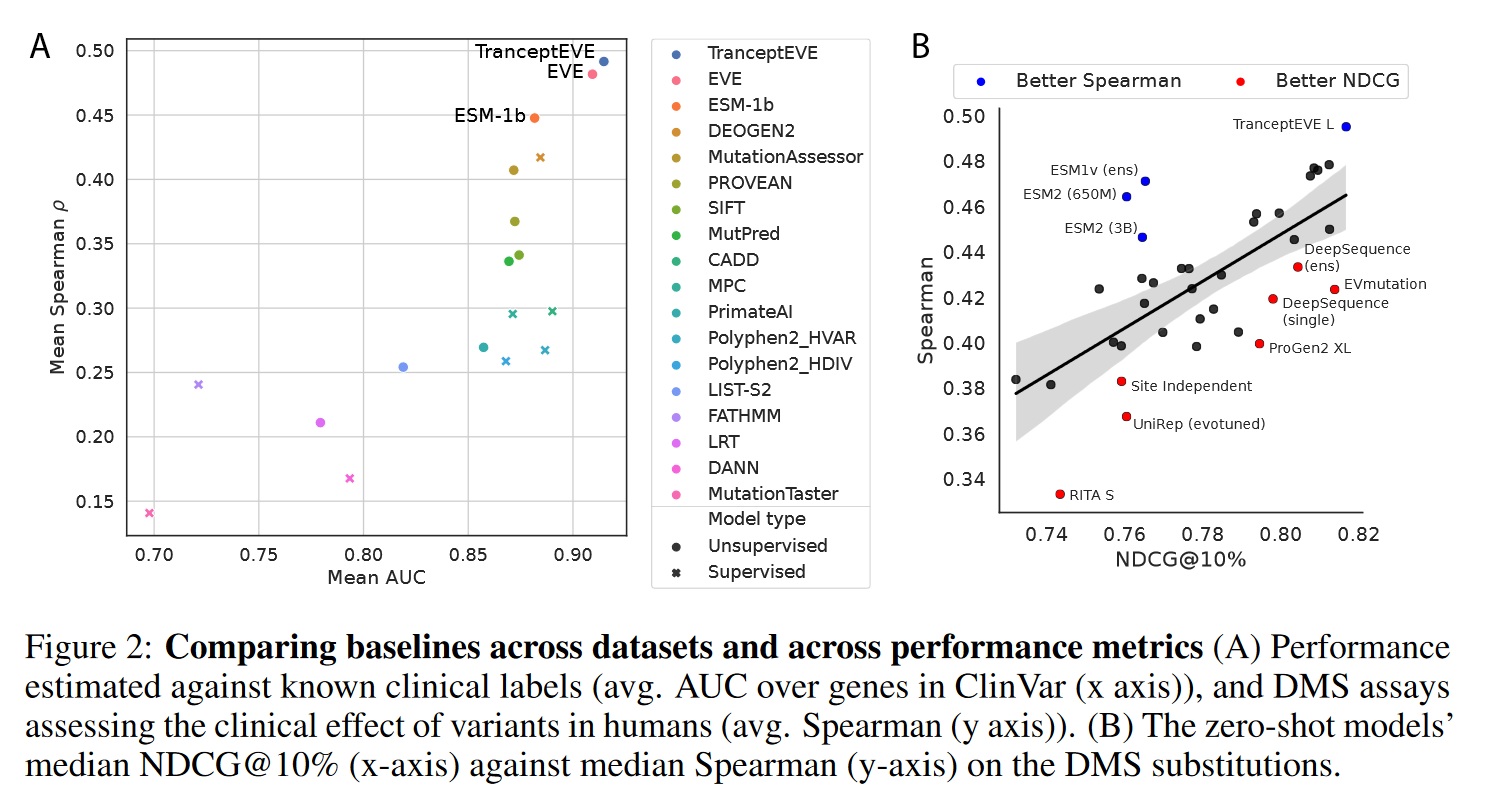
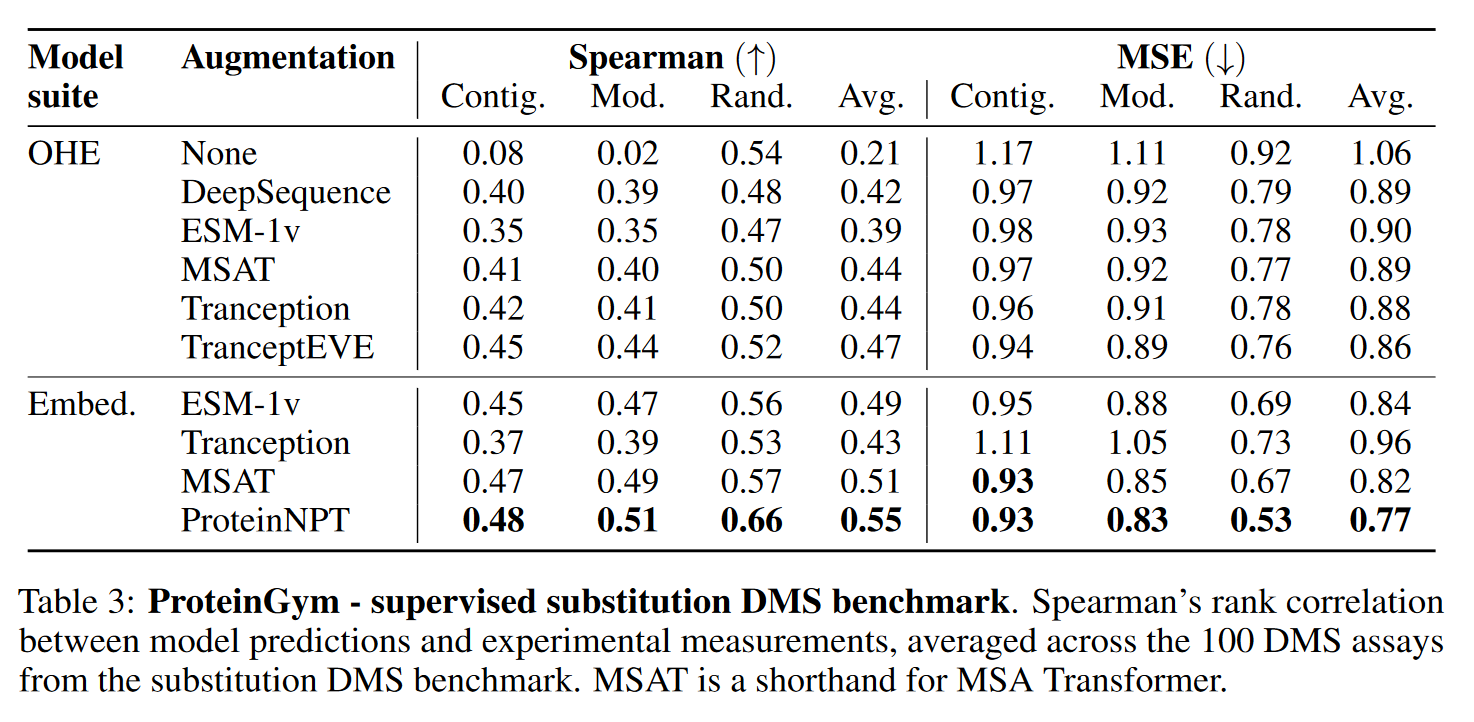
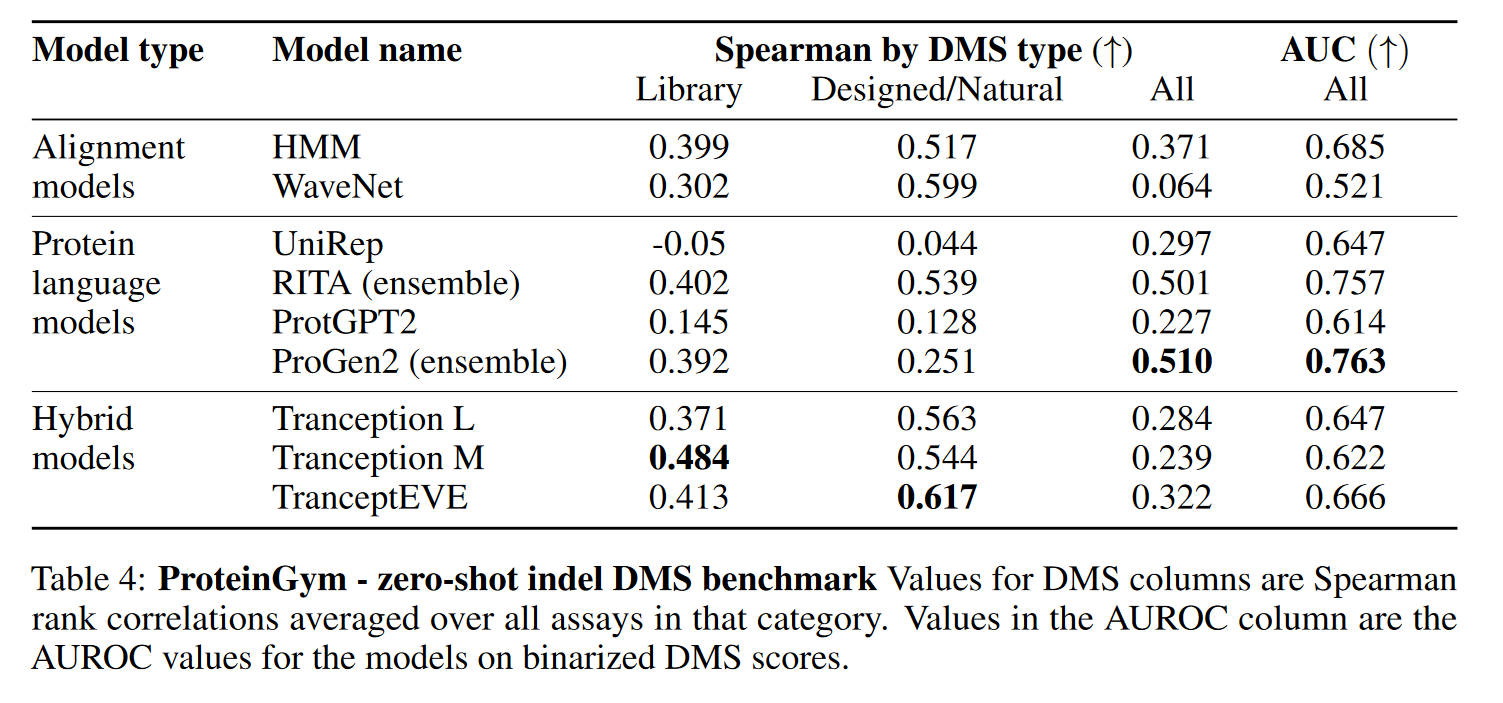